Ever wished that you could just train your chatbot in an instant? What if we told you that we’ve made it possible for you to train your chatbot in just 8 seconds?
Thanks to our relentless focus towards our customers and dedication towards solving for their challenges and needs, we’ve made this happen. Our new DocuSense technology allows you to do away with manually uploading FAQs and just upload a document instead. With DocuSense assimilating 12 pages of information every 8 seconds, you can have your bot trained in no time.
The fastest way to train your chatbot - DocuSense
DocuSense is our new offering that allows you to just simply upload a document that has all the relevant information that the chatbot needs to look up and respond to the user. These could be policy documents, knowledge-base articles, technical documentation or anything that has relevant content and information for the user. That’s it. No fancy bells and whistles, just sheer dedication towards our goal of usable intelligence.
You can even upload multiple documents, categorize them to control the searching, and configure the manner in which the responses are sent out to the users. Also, they come packaged in our own native Document Viewer that will take you to the relevant section so that you get the information you need with the complete context.
DocuSense can be enabled for your bot by accessing the feature from the Cognitive Search option within Smart Response section.
Why DocuSense?
We’ve worked with a number of customers who have used our NLP and ML capabilities via our Smart Response set of capabilities. Their main pain point has been the manual chatbot training of the FAQs in the system. Although, this has significant benefits in terms of tweaking the chatbot’s responsiveness and efficiency to suit your exact requirements, it does involve some work and monitoring on an on-going basis as well.
We went back to the drawing board and put on our thinking hats to drill down on some common use-cases and figure out how we can make the experience as effortless and seamless as possible.
With continuous research, proofs-of-concept, testing, and refinements, we came up with a way to provide an alternative option to have an efficient, responsive chatbot with minimal effort. We came up with DocuSense.
Diving deeper into bot training
Now that you have a basic understanding of the feature, let’s take a closer look at some of the details around it –
The Cognitive Search workflow is where you would upload the documents that you want to load and train the chatbot with. During the upload, you can provide the title that would be used in the Viewer. Once the document is uploaded, it hardly takes a few minutes for the bot to process and index the document, after which the chatbot is ready to respond with relevant results from the document.
The documents can also be categorized and you can use specific nodes to restrict the search to a particular category of documents.
If the document gets updated, you can use the workflow and select the edit option to re-upload the document.
You can also have an external link to the document that points to the live version of the document that can be accessed from the viewer.
As highlighted before, the solution comes packaged with our Document Viewer, which allows the users to go through the complete document in context.
For each result, the relevant section of the result in the document will be highlighted. Also, the user would be navigated to the page where the matching section was found.
It also has standard controls for navigating the document, scrolling and zooming options.

A number of configuration options are provided for you to customize the experience as well as the lookup behavior.
Some things that can be customized are — number of matches that are shown to the user, prompt for showing the results, how the response is presented (which content elements are to be shown). This also includes Document Name and the link to open the Document Viewer.
You can also select the color that would be used to highlight the match that was found in the document.
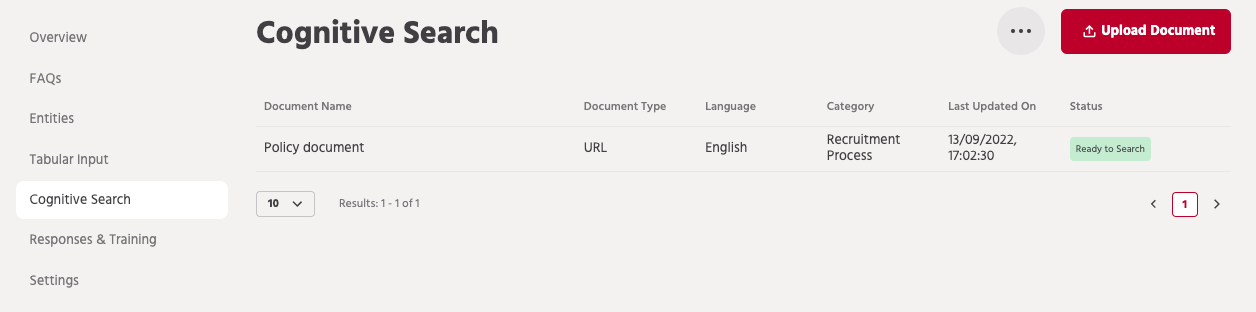
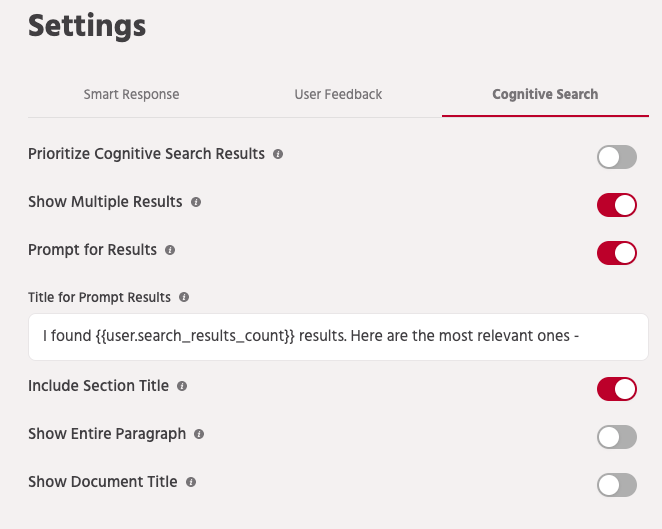
What key phrases do you need to train your chatbot with?
3 key phrases are very important when it comes to bot training. These 3 terms are:
Utterance, in simple words, would be predicting what your user might ask/say to your chatbot. It might include greetings, common queries, etc.
An intent usually represents what the customer is looking for from your chatbot. A good example would be, a person looking for the latest news from a news website. If they say "show me today's news", the chatbot collects the user's intent which is to read today's trending headlines. Intents are usually a combination of a verb and a noun, such as "ShowNews''.
Entities are keywords or values that you can extract from a conversation that help make their intent more clear. A common user query could be "How to enrol for a chatbot building course?" Here the user might find the exact course, or they might also go on to try out a course similar to that what they were initially looking for.
How can you use Engati’s all new eSense GPT?
We have upgraded and simplified the whole bot training process, with Engati’s new and advanced integration ‘eSenseGPT’ has the potential to answer a variety of questions that are relevant to the data or the document entered in it. Just enter your data in the form of PDF, Google Docs, or a website link into the ‘eSenseGPT’ integration and it will provide prompt responses to any complex queries about procedures, guidelines, policies, etc.
%20copy.webp)
How to train ai chatbot?
Engati provides you with the flexibility to train your AI chatbot how you prefer. For simpler requests, you can train with:
Let's start with FAQs, or Frequently Asked Questions. Training with this option works best for more transactional inquiries, training with FAQs. To configure FAQs, click on the Build tab in the left panel and select the FAQ option.
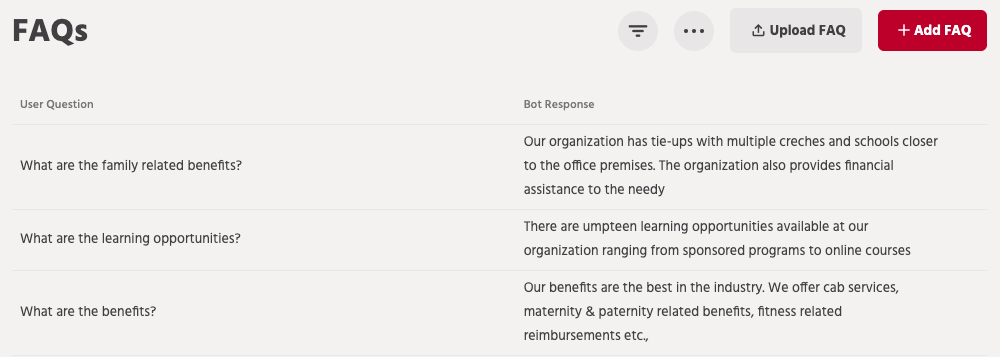
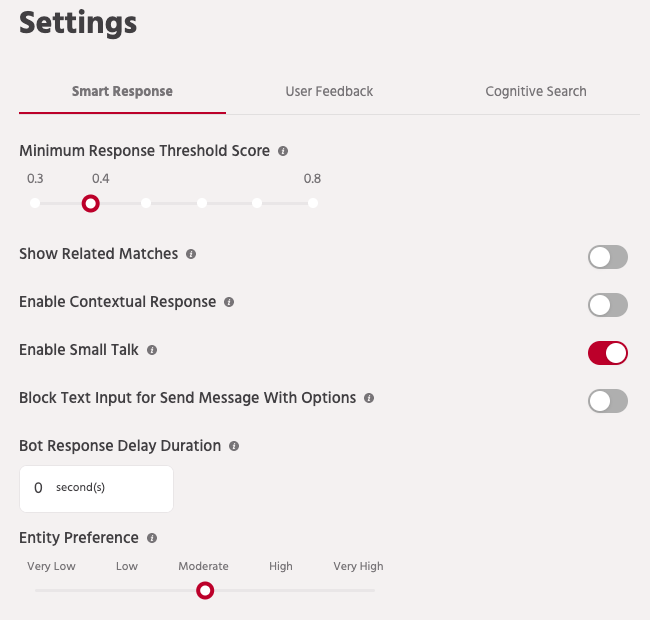
There are two ways to add FAQs to your bot. You can either add one FAQ at a time, or you can add multiple FAQs.
One FAQ at a time
If the questions asked by your customers are specific, you can add FAQs one-by-one.
Click on Add FAQ button on the FAQ page and add:
Categories: To help you segment relevant queries together and also helps you apply an FAQ filter in a given path. A simple example can be of an organization that has multiple departments lets say finance, marketing, support, and operations. You can segregate all your queries in these categories. While using the support path you can limit the FAQs to support category.
Language: For multiple languages
Question: This is the query that your customers ask the bot. You can add multiple questions and variations for a given response. This covers the variation that may occur when different users ask the same questions.
Entities: When a group of values leads to the same answer you can tag entities within the FAQ rather than creating separate FAQs for all the variables.
Multiple FAQs
If you have a large number of incoming questions and many categories, you can simply upload a formatted PDF to CSV file.
All you have to do is click on Add FAQ button on the FAQ page.
Use the FAQ CSV file upload mechanism to instantly train the chatbots with multiple FAQ. This is a structured document. The detailed usage instructions for which are provided below.
Coming back to entities, they're essentially data points or values that you can extract from a conversation or query. This allows you to customize what kind of information you are collecting, how you want to associate it and even add your own custom set of values, if needed. Also when a group of values leads to the same answer you can use entities rather than creating FAQs for all the variables.
For Instance, the enrolment procedure for all the courses in an institute is the same. A common user query maybe “How can I enroll for Artificial Intelligence course?” similarly there can be n number of queries in the same context for different courses. Here rather than creating different FAQs for each subject you can create an entity set with custom values and add all the course names in it and it will give relevant answers each time the query is triggered.
Creating an entity
Click on Build and select Entity section to manage entities. You will see an option to “Add an entity” which launches this popup box as shown below in order for you to create an entity type for use in the conversations.
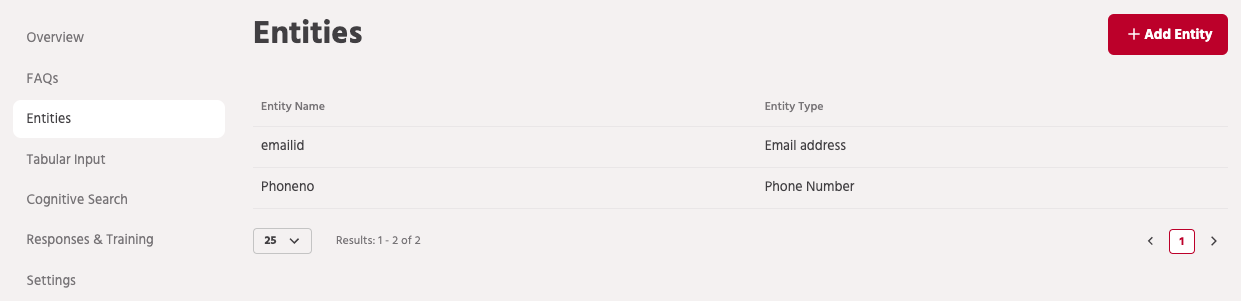
You can give any name to your entity related to the information you are collecting, for e.g – booking_date, courses, products, etc. The only restriction being you cannot add a space or a slash character in the name. Also, it cannot be the same as a built-in entity type like – number, date, time, etc. The name is important because this will be used extensively for using the extracted values in the conversation.
You can define multiple entities of the same type in cases where the usage is different or we want different values to be associated there. For example – For a travel bot, I can have booking_date and travel_date as separate entities although both are of Date and Time types.
Say for example, you have an education bot for helping students with course information. It’s far too time-consuming to write FAQs for each set of information. Instead you can create custom entities with tabular input. You can create custom entity sets for courses, fees, start date, prerequisites, etc.
Before you start, make sure that your data table is ready in a file with the structure as explained below. Each row should represent one entity from the set that the chatbot is expected to provide details for.
Each column has to represent the various aspects of which users would want to query the chatbot.
First step is to click on Build and select data source.
Second step is to upload the file which has tabular data for entities and their information in each column. Supported file formats are .xls, .xlsx and .csv. A sample file is also attached here for reference.
Download Sample data Source here.
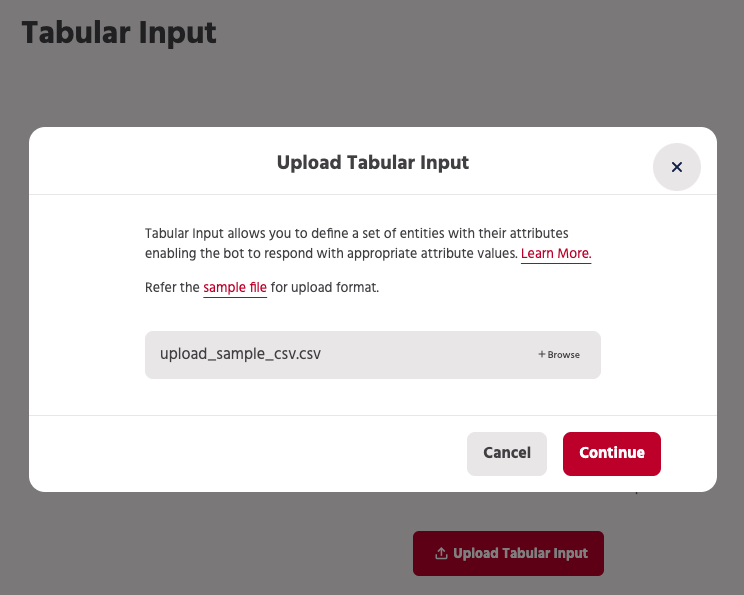
Name the data source and the Entity name to be used for these custom value.
What are Synonyms and stopwords?
Synonyms
Synonyms are alternate words to denote the same object or action. From a bot and context relevancy, typical use cases involve your domain-specific synonyms. It could also be used for cases of common misspellings, abbreviations, and similar uses.
To configure Synonyms, go to Build and click FAQ in the advanced menu. Then click on "Configure Synonyms" to configure these options.
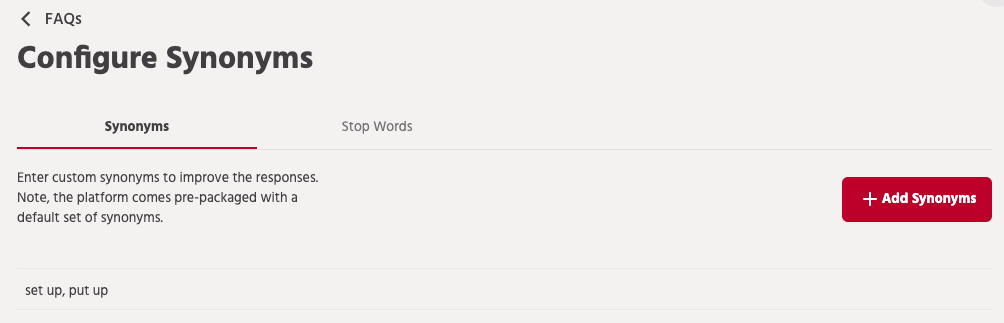
Engati has a pre-configured set of Synonyms for English. This capability allows you to add to that list for your bot.
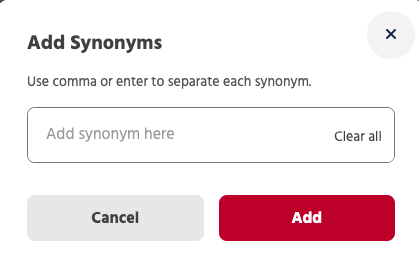
You can click on “Add” and add synonyms to your bot.
You can add more than one synonym by pressing the tab/enter key after each entry. Our system finds matching synonyms and adds to your list, you can remove these if you don’t find it relevant.
Engati also lets you add synonyms for any language other than English in the exact same manner as you do it for English. All you have to do is select the other language in question while adding a new Synonym.
Stopwords
Stopwords are a set of commonly used words in a language. Examples of stop words in English are “a”, “the”, “is”, “are” and etc. The intuition behind using stop words is that, by removing low information words from the text, we can focus on the important words instead of the actual NLP.
Engati comes with a list of predefined Stopwords but it’s not uncommon to see some domain or use case-specific stop words. As an example, if the chatbot is to answer queries about various features of a product, it might be prudent to include that particular product name itself as one of the Stopwords.
Click on the Stopwords tab to add or remove stopwords. These words are ignored by NLP when matching FAQs to relevant responses. There are many stopwords preconfigured for a bot.
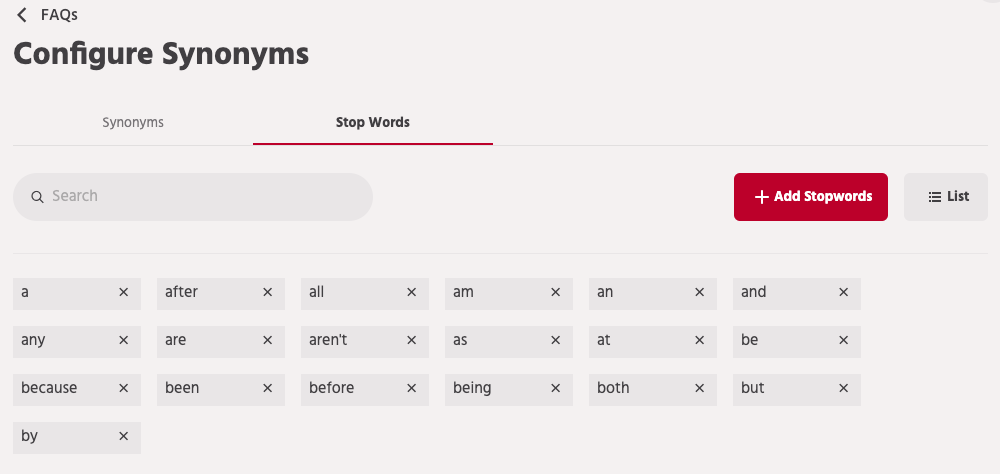
You can add more than one stopword in a go by separating them with a comma (,).
Also do check out this article about synonyms and stopwatches for more.
NLP helps you in bot training & makes it more intelligent
That basically means adding to its repository of responses based on real-world questions that the users ask. NLP will help you deliver a higher level of interaction and engagement with your user settings and further reduce any transfers to humans. The need for humans will always remain – as a “Talk to an expert” path, as bots become more advanced in its speed and accuracy of understanding text and speech.
At the broadest level of NLP, the utilisation and fits have evolved over time. We still are progressing on Generation 1 and 2 bots, simple Q&A bots or bots that integrate to back-end platforms to transact.
Evolution 1
At the very beginning, we used NLP models and still use it for spam filtering. We started using NLP algorithms in another area that is Speech tagging. It was felt that we could solve these issues by using simple interpretative essentials models. The need for more complex deep learning algorithms was felt since the basic interoperability algorithms only took us so far.
The main issue in normative translation using simplified models was the extent we use intents based on colloquialism, contextual sensitivity, political correctness, beating around the bush, idioms, sayings interlaced with oxymorons and irony in our daily communication. Why should our conversation with a bot be any different?
Evolution 2
Our base FAQ algorithms used for scoring words, extracting context and intent would be inadequate. It will not make the FAQ chatbot more intelligent. The average summation of a bag of words model and using vector science will only take us so far along the accuracy curve. To further improve we try n-grams or shingles. N-grams is a statistical probability determinant model for predicting the next item in a sequence of words. An n-grams approach struggled with the dimensionality of interpretations. Even Markov models were inadequate to cater to this level of vagaries and needs. The N-grams approach is what has been described in blogs above where word scoring and an average summation led to a vector for sentences to compare with pre-stored Q&A formats.
Evolution 3
The main issue with the approach was the linearity of words and the vector science behind it. With distinct words, all vectors representing single words would be equidistant. It lacked semantic information. If we migrate from the bag of words model approach then it will help increase the accuracy of the response. This model allowed for words similarity pairs if used in the same context.
Evolution 4
A further evolution was the use of semantic matching through a global word to word co-occurrence matching matrix. The base algorithm relied on the difference vectors between words. Further multiplied by the context word, if equal to the ratio of their co-occurrence probabilities.
Evolution 5
Though the use of the global word to word co-occurrence matrix in Evolution 4 increased the accuracy of base model predictions, long and short term dependency determination was still found to be lacking.
This evolution called for the application of Recurrent Neural Networks (RNNs) which fed text data in a sequence to the network. The RNN application was an improvement since it handled local temporal dependencies quite effectively. The problem arose in figuring out long sentence forms. To address the issue of long form sentences, researchers designed an algorithm called Long-short term memory (LSTM). This algorithm introduces a memory cell that stores long term dependencies of the long form sentence being processed.
Think of it as an indicator score that updates based on every word context to store the overall vector in long form sentences. This allowed for weightage based on binary science that allowed for the network to forget or weigh it higher for relevance, making the RNN further optimized for relevance.
Evolution 6
Researchers were advancing the use of RNN and LSTM techniques for word context and intent. And another parallel application was producing success and results. The name of the approach is Convolutional Neural Networks or CNNs. Would it be possible to use a CNN in an NLP solution framework to further enhance accuracy? It sure did seem so.
Consider a 2D model that image applications use to solve for a small segment of an image to do an ID filter. Could we use the technique for linear sentences of words to predict context and intent?
It sure did seem so. ID-based CNNs were more accurate than RNNs. Multiple CNNs working on a construct could create a feature map. They could learn from the features that appear the most often. CNNs still remain a work in progress with promising results on the default Q&A mechanisms used in bots for lightweight determinations of questions and the answers that match the search intent and context.
What to look forward to?
This is just the beginning of a journey and we’re already busy with the next set of enhancements — Support for complex document structures and elements, adding support for other languages, and ongoing efforts to refine the Cognitive Search efficacy.
This leads to our efforts around Knowledge Management where the bot learns from various knowledge sources and intelligently determines which is the best and most relevant source for a user query, given the context.
Here's a good article on how to write knowledge base articles!
Interested?
Reach out to us at contact@engati.com to get a glimpse of our bleeding edge technology and learn how it can help you for your use-case.