What is an algorithm?
In the most general sense, an algorithm is a series of instructions telling a computer how to transform a set of facts about the world into useful information. The facts are data, and the useful information is knowledge for people, instructions for machines or input for yet another algorithm. There are many common examples of algorithms, from sorting sets of numbers to finding routes through maps to displaying information on a screen.
To get a feel for the concept of algorithms, think about getting dressed in the morning. Few people give it a second thought. But how would you write down your process or tell a 5-year-old your approach? Answering these questions in a detailed way yields an algorithm.
In mathematics and computer science, an algorithm is a finite sequence of well-defined, computer-implementable instructions, typically to solve a class of specific problems or to perform a computation.
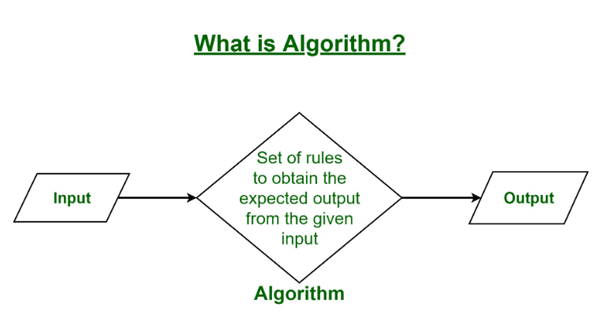
What are the characteristics of an algorithm?
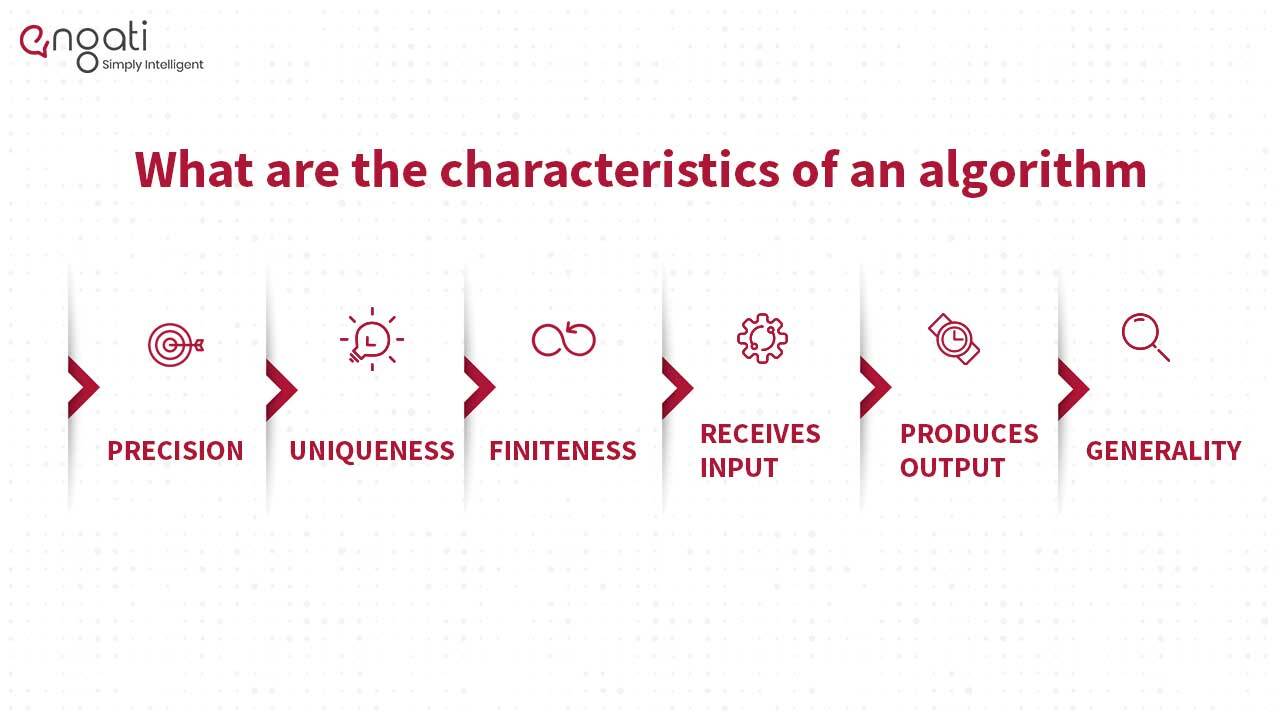
- Precision – the steps are precisely stated.
- Uniqueness – results of each step are uniquely defined and only depend on the input and the result of the preceding steps.
- Finiteness – the algorithm stops after a finite number of instructions are executed.
- Input – the algorithm receives input.
- Output – the algorithm produces output.
- Generality – the algorithm applies to a set of inputs.
How do computer algorithms work?
Computer algorithms work via input and output. They take the input and apply each step of the algorithm to that information to generate an output.
For example, a search engine is an algorithm that takes a search query as an input and searches its database for items relevant to the words in the query. It then outputs the results.
You can easily visualize algorithms as a flowchart. The input leads to steps and questions that need handling in order. When each section of the flowchart is completed, the generated result is the output.
How to design an algorithm?
.jpg)
1. Obtain a description of the problem
This step is much more difficult than it appears. You first have to create a description of the problem. It's quite common for a problem description to suffer from one or more of the following types of defects:
- The description relies on unstated assumptions,
- The description is ambiguous,
- The description is incomplete,
- Or the description has internal contradictions.
These defects are rarely due to carelessness. Instead, they are due to the fact that natural languages are rather imprecise. Part of your responsibility is to identify defects in the description of a problem and to work with the client to remedy those defects.
2. Analyze the problem
The purpose of this step is to determine both the starting and ending points for solving the problem. This process is analogous to a mathematician determining what is given and what must be proven. A good problem description makes it easier to perform this step.
When determining the starting point, we should start by seeking answers to the following questions:
- What data are available?
- Where is that data?
- What formulas pertain to the problem?
- What rules exist for working with the data?
- What relationships exist among the data values?
When determining the ending point, we need to describe the characteristics of a solution. In other words, how will we know when we're done? Asking the following questions often helps to determine the ending point:
- What new facts will we have?
- What items will have changed?
- What changes will have been made to those items?
- What things will no longer exist?
3. Develop a high-level algorithm
An algorithm is a plan for solving a problem but plans come in several levels of detail. It's usually better to start with a high-level algorithm that includes the major part of a solution but leaves the details until later.
4. Refine the algorithm by adding more detail.
A high-level algorithm shows the major steps that need to be followed to solve a problem. Now we need to add details to these steps, but how much detail should we add? The answer to this question really depends on the situation. We have to consider who (or what) is going to implement the algorithm and how much that person (or thing) already knows how to do.
When our goal is to develop algorithms that will lead to computer programs, we need to consider the capabilities of the computer and provide enough detail so that someone else could use our algorithm to write a computer program that follows the steps in our algorithm. Remember, when in doubt, or when you are learning, it is better to have too much detail than to have too little.
For larger, more complex problems, it is common to go through this process several times, developing intermediate-level algorithms as we go. Each time, we add more detail to the previous algorithm, stopping when we see no benefit to further refinement. This technique of gradually working from a high level to a detailed algorithm is often called stepwise refinement.
Stepwise refinement is a process for developing a detailed algorithm by gradually adding detail to a high-level algorithm.
5. Review the algorithm.
The final step is to review the algorithm. What are we looking for? First, we need to work through the algorithm step by step to determine whether or not it will solve the original problem. Once we are satisfied that the algorithm does provide a solution to the problem, we start to look for other things. The following questions are typical of ones that should be asked whenever we review an algorithm.
Asking these questions and seeking their answers is a good way to develop skills that can be applied to the next problem:
- Does this algorithm solve a very specific problem or does it solve a more general problem? If it solves a very specific problem, should it be generalized?
- Can this algorithm be simplified?
- Is this solution similar to the solution to another problem? How are they alike? How are they different?