What is Natural Language Processing?
Natural Language Processing (NLP) is a branch of Artificial Intelligence (AI) that helps computers understand, interpret and manipulate human language.
It uses computational linguistics (ruled-based modelling of natural language) with machine learning, statistical, and deep learning models to analyze natural language and understand the actual meaning of text or voice data. It even understands the speaker’s or writer’s sentiment and intent.
It isn’t a new science, but has been advancing at a fast pace due to the availability of big data, increasingly effective algorithms, and the heightened interest in human-to-machine interaction and communication. NLP programming combines the fields of linguistics and computer science to decipher language structure and guidelines to comprehend, break down, and separate significant details from text and speech. It automates the translation process between computers and humans by manipulating unstructured data (words) in the context of a specific task (conversation).
Natural language processing aims to enhance the way computers understand human text and speech and translate languages with logic-based learning. As computers can only understand and decipher programs and follow instructions, NLP works around making the process of understanding and reading languages much more efficient.
NLP uses artificial intelligence and machine learning, along with computational linguistics to process text and voice data, derive meaning, figure out intent and sentiment, and form a response or input. Computers cannot understand or interpret text and words the way humans do, as they communicate in 1s and 0s. Hence, NLP enables computers to understand, emulate and respond intelligently to humans.
Why is Natural Language Processing important?
The main reason why Natural Language Processing is extremely important is that it helps analyze and make sense of vast volumes of data. It helps process text as well as voice data, understands sentiments and intents and even helps derive critical insights from the data.
Natural language is extremely complex and the data is largely unstructured. Textual data contains misspellings, abbreviations, missing punctuations, while voice-based data has the issue of regional accents, mumbling, stuttering, etc. When speaking or writing, different languages have different grammar and syntax rules. We even end up using words and phrases from other languages quite often.
Natural language processing (NLP) is of critical importance because it helps structure this unstructured data and reduce the ambiguity in natural language.
It even helps businesses organize their data in a manner that works more effectively for them.
-min.jpg)
How does Natural Language Processing (NLP) work?
NLP tools convert text into something a machine can understand using text vectorization, and then machine learning algorithms are fed training data and expected outputs (tags) to train machines to make associations between a specific input and its corresponding output. Before making predictions for unseen data, machines use statistical analysis methods to build their own "knowledge bank" and determine which features best represent the texts.
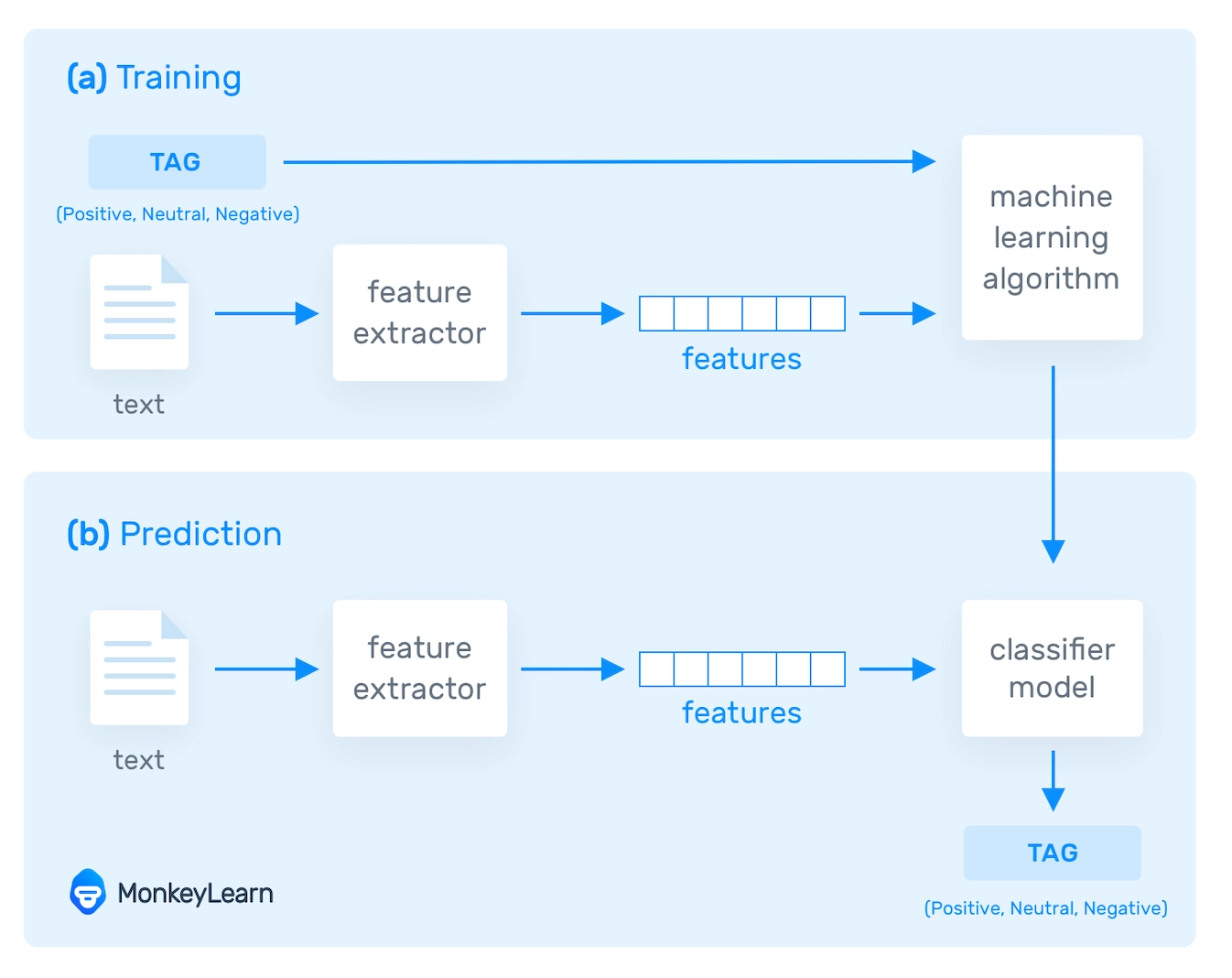
The more data fed to these NLP algorithms, the more accurate the text analysis models will be in the end. Sentiment analysis (shown in the graph above) is a popular NLP task in which machine learning models are trained to classify text based on the polarity of opinion (positive, negative, neutral, and everywhere in between).
The ability of machine learning models to learn on their own, without the need for manual rules, is their most significant advantage. All you need is a set of relevant training data with a few examples for the tags you want to look at. You can also use advanced deep learning algorithms to chain together multiple natural language processing tasks, such as sentiment analysis, keyword extraction, topic classification, intent detection, and more, to achieve super fine-grained results.
NLP software performs three main processes at a basic level:
- It begins by breaking down the language (voice or text) into its constituent parts.
- It then tries to figure out how these bits of data are related to one another.
- Finally, it attempts to make sense of these connections.
Of course, there are many more steps in each of these processes. Programming, algorithms, and statistics, as well as linguistic knowledge, are all required.
What are the various text preprocessing steps in Natural Language Processing (NLP)?
Data preprocessing is an important step in building a Machine Learning model, and the results are dependent on how well the data has been preprocessed.
Text preprocessing is the first step in the NLP model-building process.
The following are the various text preprocessing steps:
Segmentation
You must first break down the entire document into individual sentences. This can be accomplished by segmenting the article and its punctuation, such as full stops and commas. We can suppose that each English sentence represents a distinct thinking or idea. Writing a program to understand a single sentence will be far easier than understanding a whole paragraph. Splitting sentences apart anytime you see a punctuation mark is a straightforward way to code a Sentence Segmentation model. Modern NLP pipelines, on the other hand, frequently employ more advanced algorithms that operate even when a page isn't well-formatted.
For example:
A dog is barking, and the caretaker is scared of him.
"A dog is barking" and "The caretaker is scared of him" will become two segments.
Word Tokenizing
You must get the terms in a sentence and explain them individually to our system for the algorithm to grasp them. As a result, you deconstruct your statement into its constituent words and save them. This is referred to as tokenizing, and each world is referred to as a token. We may now process each sentence individually after splitting our document into sentences.
Breaking this statement into individual words or tokens is the next stage in our pipeline. This is referred to as tokenization. The result is as follows:
"A" "dog" "is" "barking" "and" "the" "caretaker" "is" "scared" "of" "him"
Removing Stop Words
Then we'll examine each symbol to see which part of speech corresponds to which noun, verb, adjective, and so on. Knowing what each word in the sentence does will help us figure out what the sentence is about.
And it'll look like: "Dog" "Barking" "Caretaker" "Scared" "Him"
Stemming
Stemming is the process of determining a word's Word Stem. When you add affixes to existing words, Word Stem generates new ones.
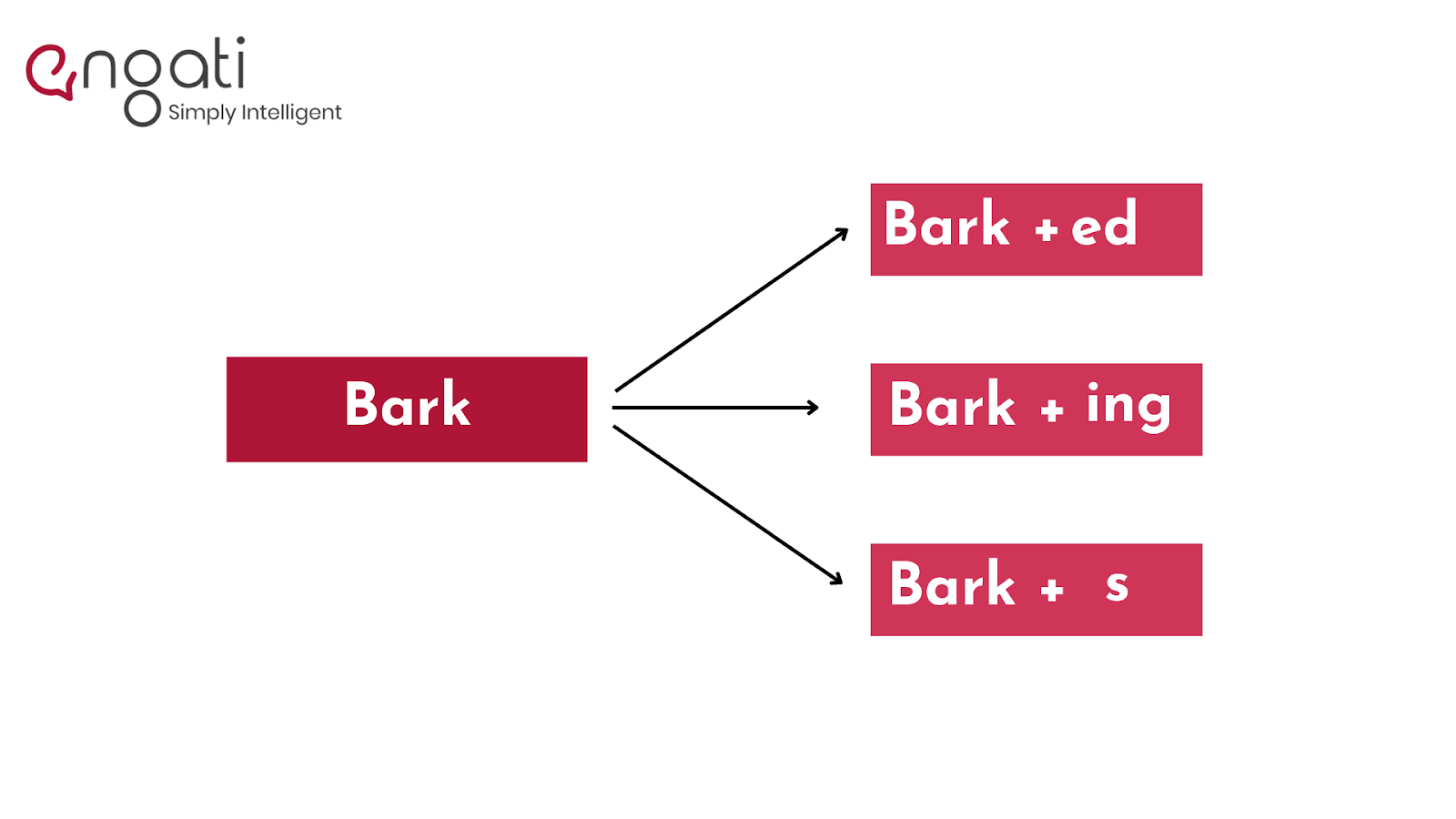
Lemmatization and Tagging the Parts of Speech
Lemmatization is usually accomplished by using a look-up table containing lemma forms of words depending on their part of speech, as well as some unique rules to deal with terms you've never seen before. The procedure for obtaining a word's Root Stem. The new base form of a word that is in the dictionary and from which the word is developed is given by Root Stem. You may also determine the root words for other terms based on tense, mood, gender, and other factors.
By adding these tags to our words, you must now explain the concept of nouns, verbs, articles, and other parts of speech to the machine. This is known as 'part of.'
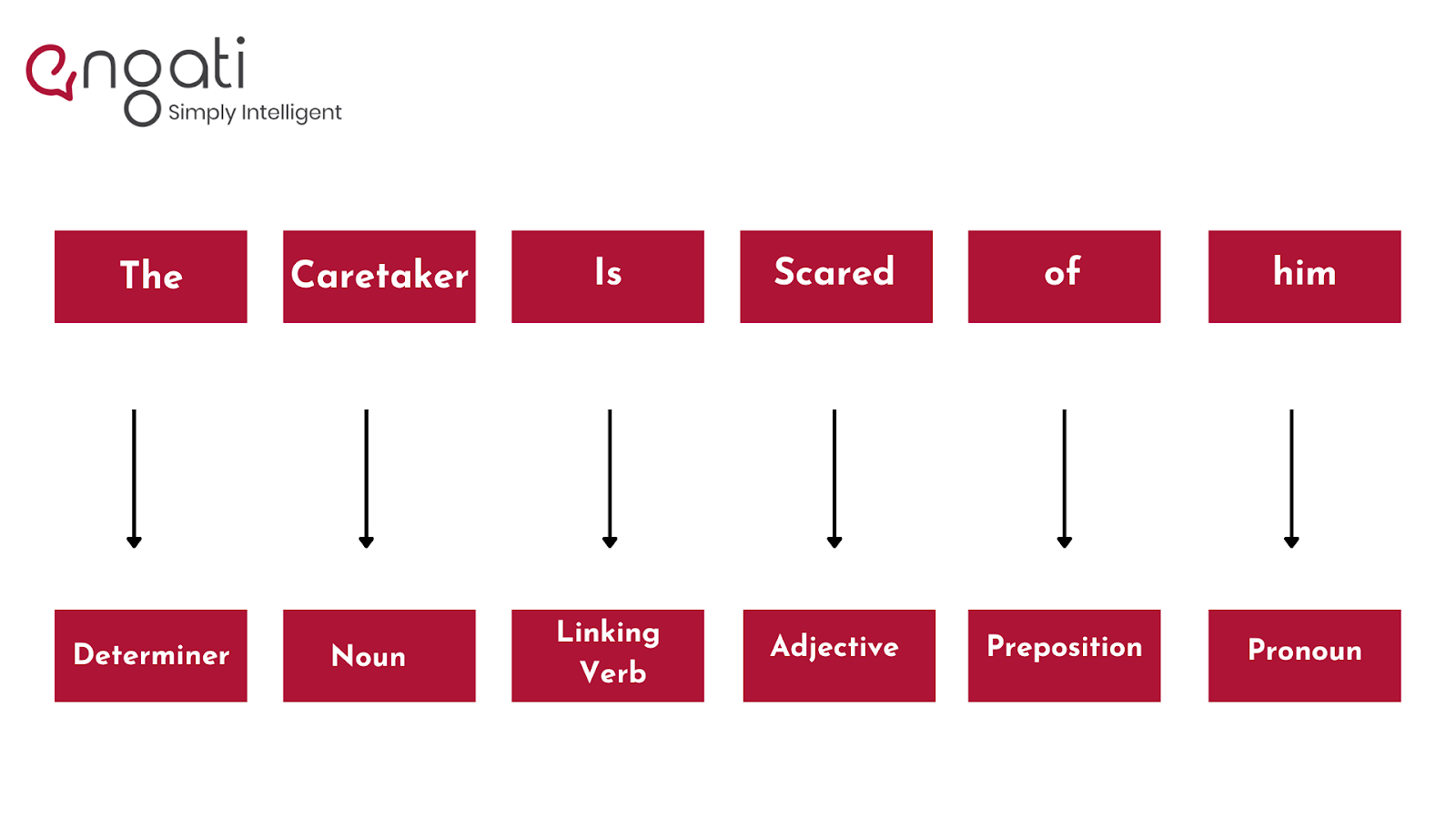
Named Entity Tagging
Next, teach your machine to recognize pop-culture allusions and ordinary names by highlighting movie titles, important people or places, and so on that may appear in the document. This is accomplished by dividing the words into subcategories. This aids in the discovery of any keywords within a sentence. Person, place, monetary value, amount, organization, and movie are the subcategories.
After you've completed the preprocessing procedures, you'll use a machine learning method such as Naive Bayes to develop your NLP application.
Dependency Parsing
The following step is to determine how all of the words in our phrases are related to one another. Dependency parsing is the term for this.
The goal is to create a tree that gives each word in the text a single parent word. The key verb in the phrase will be the tree's root.
Applications of NLP
NLP is one of the methods for humanizing robots and reducing the demand for manpower. Speech-related tasks and human contact have been automated as a result. NLP has a variety of applications, including:
Tools like Google Translate and Amazon Translate use natural language processing to translate sentences from one language to another.
Chatbots: Chatbots are automated responses to popular questions that may be found on most websites.
Virtual Assistants: Virtual assistants such as Siri, Cortana, Google Home, Alexa, and others can not only converse with you but also understand your requests.
Targeted Advertising: Have you ever discussed a product or service with a friend or just googled something and then started seeing advertisements for it? This is known as tailored advertising, and it may help merchants earn a lot of money by allowing them to contact niche audiences at the perfect time.
Autocorrect: Autocorrect will correct any spelling errors you make; in addition, grammar checkers will assist you in writing properly.
What are the applications of Natural Language Processing?
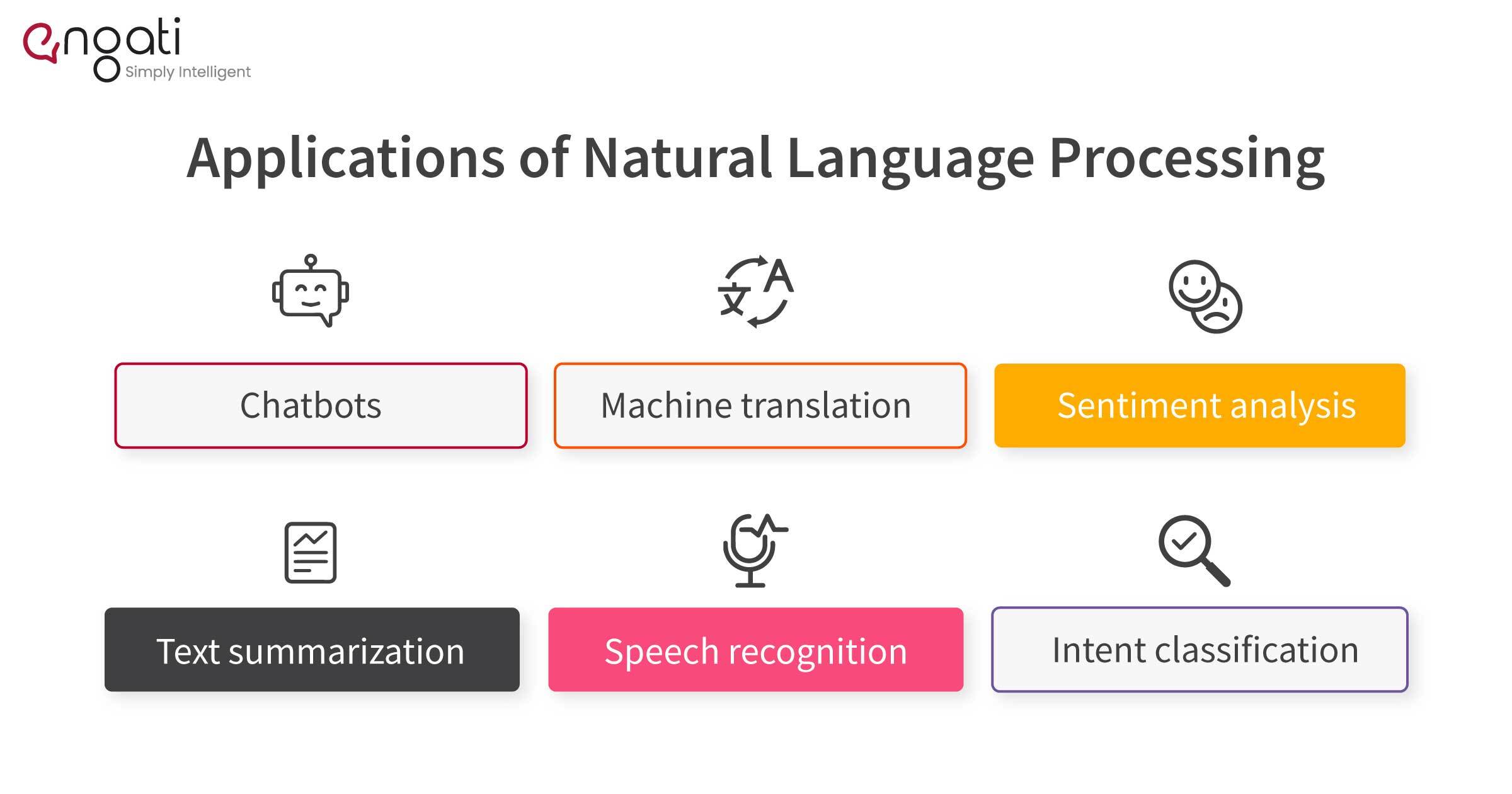
Here are a few tasks for which NLP is employed:
1. Chatbots
Chatbots are used to automate customer support, lead generation, sales, and other functions. They use NLP to understand a user’s query and deliver an appropriate response.
2. Machine translation
This involves automating the translation of data from one language to another. Translation models can even be trained for particular domains to increase the accuracy of the translation.
3. Sentiment analysis
This is used to analyze text and detect nuances in emotions and opinions. It helps you understand how positive or negative the sentiment of the data is.
Sentiment analysis is used for market research, social research, analyzing customer feedback, understanding the Voice of the Employee, etc.
4. Text summarization
This involves generating synopses of large volumes of text by extracting the most critical and relevant information.
5. Speech recognition
This refers to using NLP to turn voice data into a machine-readable format.
6. Intent classification
NLP can be used to understand the underlying motivation and the purpose behind text data. It is very useful in customer support, marketing, sales, etc.