Contrastive learning is a technique to train a model to learn representations of say images or sentences such that similar samples are closer in the vector space, while dissimilar ones are far apart. Contrastive learning can be applied to both supervised and unsupervised data and has been shown to achieve good performance on a variety of vision and language tasks. Contrastive learning has been successfully applied to Computer Vision tasks and has now been applied to NLP tasks.
A recent paper published by Princeton University highlights one such contrastive learning technique for sentence embeddings: SimCSE: Simple Contrastive Learning of Sentence Embeddings
The biggest promise of this technique is the unsupervised learning of a pre-trained language transformer model on semantic textual similarity (STS) tasks.
How does contrastive learning in NLP work?
The idea is fairly simple - take an input sentence and predict itself in a contrastive objective, using standard dropout in the neural network layers as noise. This unsupervised technique is simple and works surprisingly well performing at par with supervised models.
A supervised learning technique using natural language inference (NLI) datasets can also be used with the entailment pairs as positive samples and hence should be learned to be closer in the vector space and contradiction pairs as negative samples and should be placed far apart in the vector space.
The following table shows the accuracy of SimCSE when compared with other ways to obtain sentence embeddings for semantic textual similarity (STS) tasks.
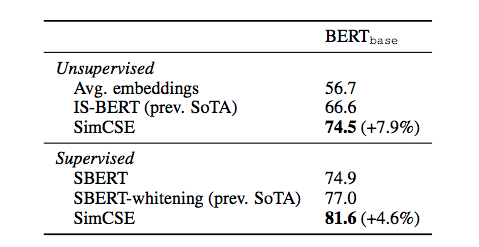
Let’s review the unsupervised technique pointed out in this paper since this holds the promise of generating trained sentence similarity models based on pre-trained language models in multiple languages without the need to create labelled datasets for training. Creating and maintaining clean labelled datasets is one of the most time consuming and error prone tasks in machine learning. Learning universal sentence representations is a tough problem in NLP and so far the only successful models have been the supervised ones trained on STS and NLI datasets.
Obtaining labelled datasets in specific domains and languages may not be an easy proposition.
As more textual data is being generated on a daily basis the need to understand and mine such textual data is becoming challenging especially due to the dependency on supervised learning.
Thus unsupervised learning is attractive.
How to automate unsupervised learning?
The following diagram (a) illustrated the simple contrastive learning technique to learn similar sentence embeddings in an unsupervised manner.

As seen above the set of input sentences are ‘Two dogs are running,' ‘A man surfing on the sea,’ and ‘A kid is on a skateboard’.
For creating positive sample pairs the same input sentence e.g. ‘Two dogs are running’ is passed into the pre-trained encoder twice and two different embeddings are obtained by applying independently sampled dropout masks. This technique of using dropout is a novel one and easy to implement.
The embeddings obtained for the other two sentences, ‘A man surfing on the sea’ and ‘A kid is on a skateboard’ are taken as negative sample pairs for the sentence embeddings generated for the positive pairs of the ‘Two dogs are running’ sentence.
Creation of labeled positive and negative sample pairs for such a task becomes very simple and can be easily automated for a large corpus.
As part of the training the embeddings for the positive sample pairs are brought closer together in the vector space and the embeddings of the negative sample pairs are pushed apart in the vector space. This trains the encoder to generate similar embeddings for similar input sentences so that the cosine similarity between these input sentences will be high.
Obtaining good representative sentence embeddings can be very useful for NLP tasks such as text classification or information retrieval. Obtaining such embeddings in an unsupervised way can scale up textual analytics in multiple domains and languages.
Is Contrastive Learning Metric Learning?
Contrastive learning is a part of metric learning used in NLP to learn the general features of a dataset without labels by teaching the model which data points are similar or different. Similarly, metric learning is also used around mapping the object from the database.
Metric Learning aims at learning a representation function that maps/clips object into an embedded space. The distance and gaps in the embedded space should take care of the objects' homogeneity/similarity, where similar objects get close and dissimilar objects at a distance. The loss function is one of the important aspects of metric learning thereby several loss functions have been developed for metic learning.
For instance, the contrastive loss navigates the objects in the metric learning from the same class to be mapped to the same point and those from different classes to be mapped to different points depending on the distance on the margin. Another case of loss function which is been used in metric learning is Triplet loss. It is a popular approach that requires the distance between the anchor sample and the positive sample to be smaller than the distance between the anchor sample and the negative sample.
What is Contrastive Self-Supervised Learning?
The major goal of self-supervised learning is to learn from the lower quality data in NLP and the goal of contrastive learning is to distinguish between similar data and dissimilar data and accordingly map it on the network. Self-supervised contrastive learning is a division of self-supervised learning that focuses on data representation. At the same time, contrastive SSL uses both positive and negative samples from the data.
Generally, we see the data representation from the self-supervised learning deployed to the algorithms for downstream tasks such as face recognition and object detection. The other benefit of contrastive SSL is that it can minimise the distance between the positive samples and ease the process of network training. More on networks in this learning is more complex and can be considered as a group of networks.
The representation is evaluated by the performance of the downstream tasks but cannot provide us with feedback on the process. Whilst, using contrastive self-supervised learning we can acquire intuition and conjectures for the efficiency of the learned representation and understand the data mapping in a constructive way. Hence, for better representation of the process and data, we use contrastive learning with self-supervised learning.
What is Contrastive Loss?
With the addition of the vector similarity and a temperature normalization factor, the contrastive loss looks unbelievingly like the softmax function.
The intuition here is that we want our similar vectors to be as close to 1 as possible, since -log(1) = 0, that’s the optimal loss. We want the negative examples to be close to 0 since any non-zero values will reduce the value of similar vectors.
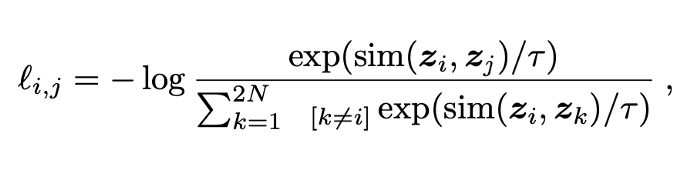
Contrastive loss can be implemented as a revised version of cross-entropy loss. And it takes the output of the network for a positive example and calculates its distance to an example of the same class and contrasts that with the distance to negative examples. In simple words, the loss is low if positive samples are encoded to similar (closer) representations and negative examples are encoded to different (farther) representations.
At the forefront of cutting edge tech
At Engati, we believe in checking out the latest research in the NLP world to understand how we can employ cutting-edge techniques to solve bigger problems faster.