What is Natural Language Generation (NLG)?
Natural Language Generation (NLG), a subcategory of Artificial Intelligence, is a process that transforms structured data into readable text. Using NLG, Businesses can generate thousands of pages of data-driven narratives in minutes using the right data in the right format.
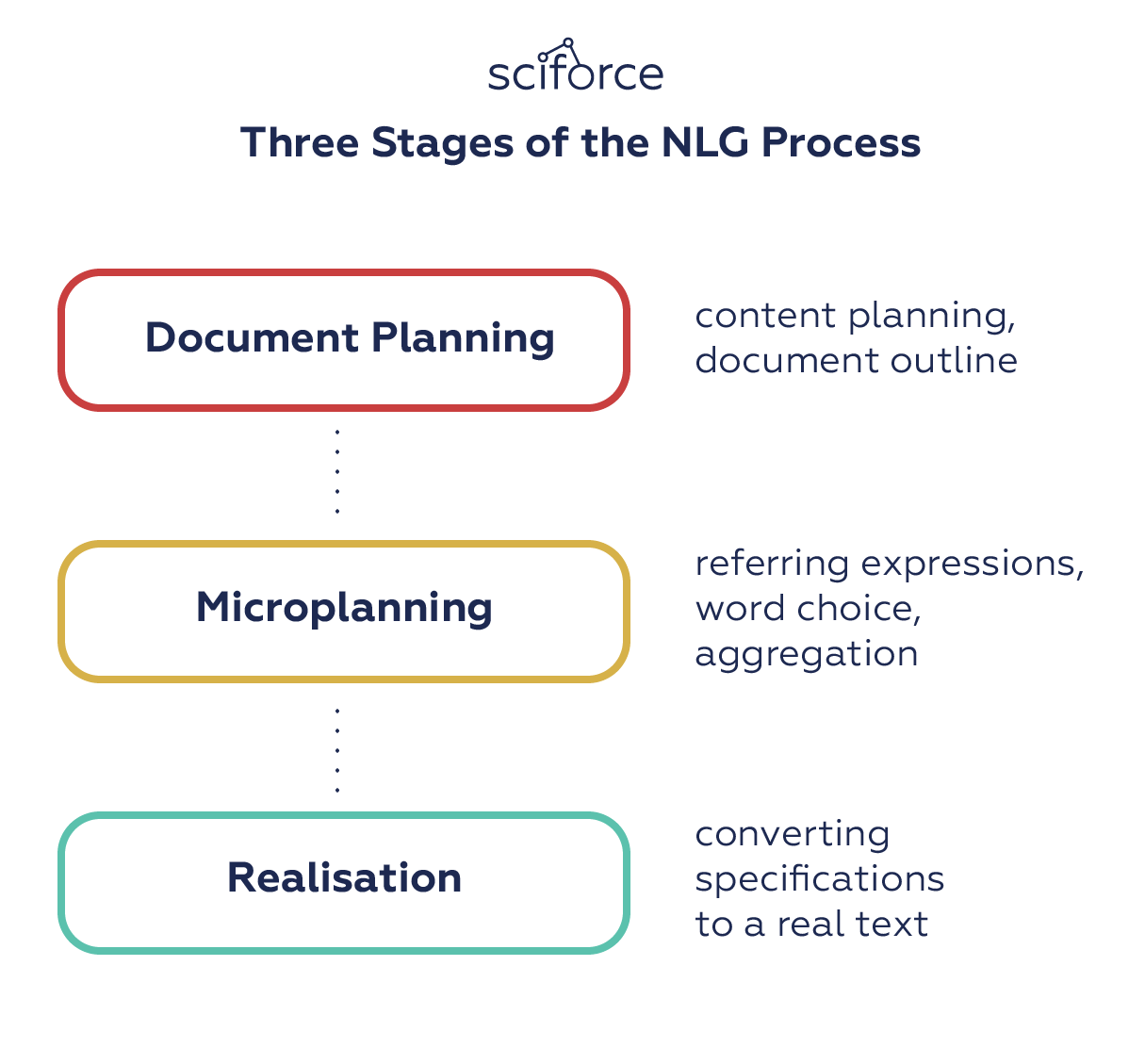
How is NLG different from NLP?
In general terms, NLG and NLU (Natural Language Understanding) are under the umbrella of Natural Language Processing- the domain that encompasses all software that interprets or produces human language, in either spoken or written form:
- NLU takes up the understanding of the data based on grammar, the context in which it was said, and decides on intent and entities.
- NLP converts text into structured data.
- NLG generates a text-based on structured data.
How does NLG work?
An automated text generation process involves 6 stages. For the sake of simplicity, we’ll explain each stage from an example of robot journalist news on a football match:
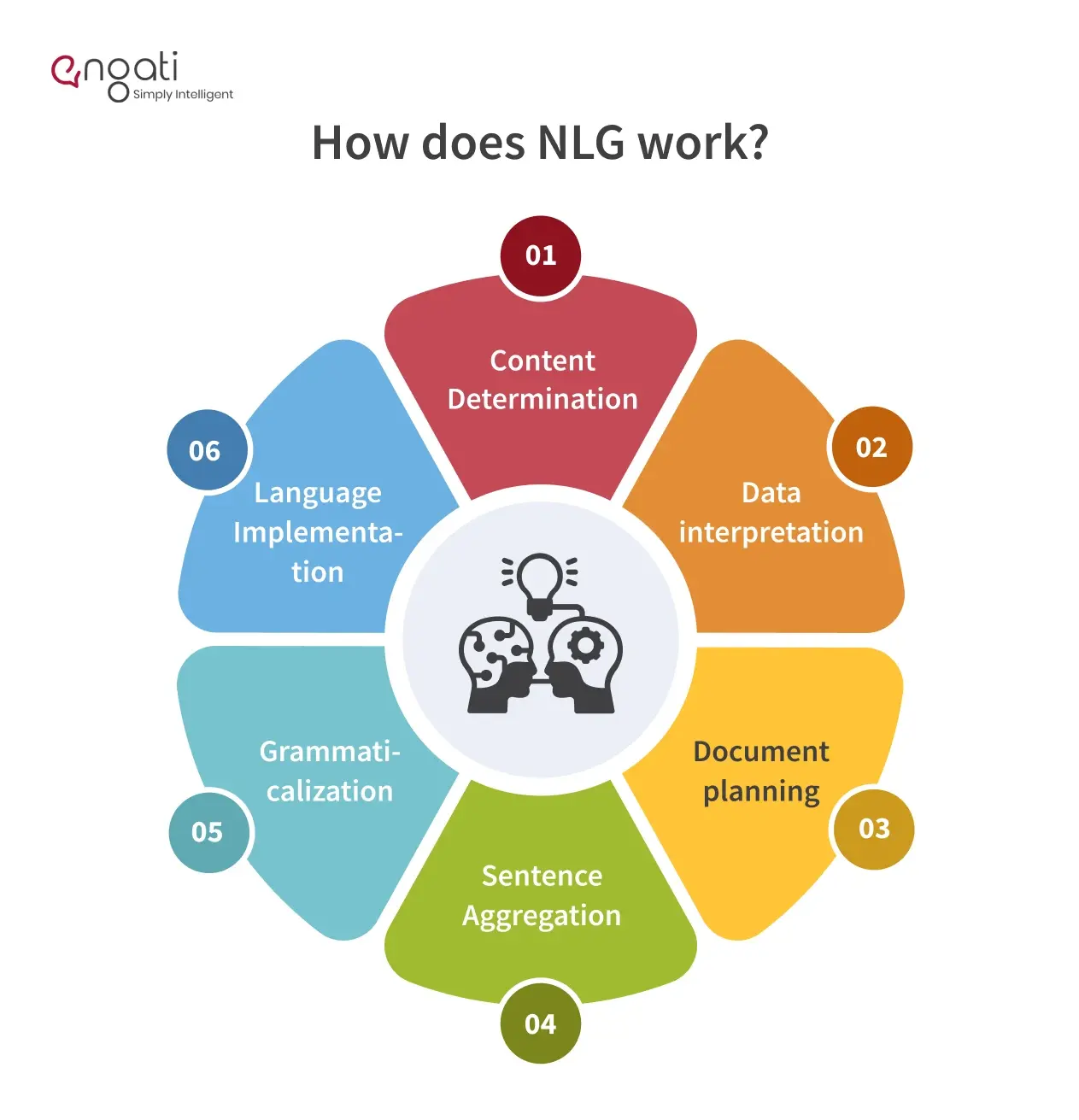
1. Content Determination
The limits of the content should be determined. The data often contains more information than necessary. In football news example, content regarding goals, cards, and penalties will be important for readers.
2. Data interpretation
The analyzed data is interpreted. Thanks to machine learning techniques, patterns can be recognized in the processed data. This is where data is put into context. For instance, information such as the winner of the match, goal scorers & assisters, minutes when goals are scored are identified in this stage.
3. Document planning
In this stage, the structures in the data are organized with the goal of creating a narrative structure and document plan. Football news generally starts with a paragraph that indicates the score of the game with a comment that describes the level of intensity and competitiveness in the game, then the writer reminds the pre-game standings of teams, describes other highlights of the game in the next paragraphs, and ends with player and coach interviews.
4. Sentence Aggregation
It is also called micro-planning, and this process is about choosing the expressions and words in each sentence for the end-user. In other words, this stage is where different sentences are aggregated in context because of their relevance. For example, below first two sentences provide different meanings. However, if the second event occurs right before half time, then these two sentences can be aggregated like the third sentence:
“[X team] maintained their lead into halftime. “
“VAR overruled a decision to award [Y team]’s [Football player Z] a penalty after replay showed [Football player T]’s apparent kick didn’t connect.”
“[X team] maintained their lead into halftime after VAR overruled a decision to award [Y team]’s [Football player Z] a penalty after replay showed [Football player T]’s apparent kick didn’t connect.”
5. Grammaticalization
The grammaticalization stage makes sure that the whole report follows the correct grammatical form, spelling, and punctuation. This includes validation of actual text according to the rules of syntax, morphology, and orthography. For instance, football games are written in the past tense.
6. Language Implementation
This stage involves inputting data into templates and ensuring that the document is output in the right format and according to the preferences of the user.
Which are the popular Natural Language Generation Models?
Even after NLG shifted from templates to the dynamic generation of sentences, it took the technology years of experimenting to achieve satisfactory results. As a part of NLP and, more generally, AI, natural language generation relies on a number of algorithms that address certain problems of creating human-like texts:
1. Markov chain
The Markov chain was one of the first algorithms used for language generation. This model predicts the next word in the sentence by using the current word and considering the relationship between each unique word to calculate the probability of the next word. In fact, you have seen them a lot in earlier versions of the smartphone keyboard where they were used to generate suggestions for the next word in the sentence.
2. Recurrent neural network (RNN)
Neural networks are models that try to mimic the operation of the human brain. RNNs pass each item of the sequence through a feedforward network and use the output of the model as input to the next item in the sequence, allowing the information in the previous step to be stored. In each iteration, the model stores the previous words encountered in its memory and calculates the probability of the next word. For each word in the dictionary, the model assigns a probability based on the previous word, selects the word with the highest probability and stores it in memory. RNN’s “memory” makes this model ideal for Natural language generation because it can remember the background of the conversation at any time. However, as the length of the sequence increases, RNNs cannot store words that were encountered remotely in the sentence and makes predictions based on only the most recent word. Due to this limitation, RNNs are unable to produce coherent long sentences.
3. LSTM
To address the problem of long-range dependencies, a variant of RNN called Long short-term memory (LSTM) was introduced. Though similar to RNN, LSTM models include a four-layer neural network. The LSTM consists of four parts: the unit, the input door, the output door and the forgotten door. These allow the RNN to remember or forget words at any time interval by adjusting the information flow of the unit. When a period is encountered, the Forgotten Gate recognizes that the context of the sentence may change and can ignore the current unit state information. This allows the network to selectively track only relevant information while also minimizing the disappearing gradient problem, which allows the model to remember information over a longer period of time.
Still, the capacity of the LSTM memory is limited to a few hundred words due to their inherently complex sequential paths from the previous unit to the current unit. The same complexity results in high computational requirements that make LSTM difficult to train or parallelize.
4. Transformer
A relatively new model was first introduced in the 2017 Google paper “Attention is all you need”, which proposes a new method called “self-attention mechanism.” The Transformer consists of a stack of encoders for processing inputs of any length and another set of decoders to output the generated sentences. In contrast to LSTM, the Transformer performs only a small, constant number of steps, while applying a self-attention mechanism that directly stimulates the relationship between all words in a sentence. Unlike previous models, the Transformer uses the representation of all words in context without having to compress all the information into a single fixed-length representation that allows the system to handle longer sentences without the skyrocketing of computational requirements.
One of the most famous examples of the Transformer for language generation is OpenAI, their GPT-2 language model. The model learns to predict the next word in a sentence by focusing on words that were previously seen in the model and related to predicting the next word. A more recent upgrade by Google, the Transformers two-way encoder representation (BERT) provides the most advanced results for various NLP tasks.