Let’s take a trip down memory lane and go back to literature class. When analyzing books, many teachers argue that it’s the thoughts of the author that matter. While us as students believed the reader’s interpretation since the reader is on the receiving end. It’s actually both that play a significant role, since art is entirely subjective.
But, what about in the case of human-technology interactions? Then, the customer’s interpretation is always going to be the winner. There’s nothing subjective there. “The customer is always right!” as the saying goes. Therefore, while developing and designing a chatbot, it’s always a matter of customer intent and entity recognition. Don’t worry, we’re going to explain what are intents and entities in a chatbot.
First, how do chatbots work?
A chatbot is a software that simulates human conversations. Users communicate using a chat interface, as if they’re texting another person. These bots interpret the words given to them and provide pre-set answers.
When training or creating a commercial chatbot, you have to feed it a lengthy set of FAQs that cover the topic you want it to answer. You can then deploy it either on a website or through other channels where customers are more likely to reach out with their queries.
Now, here’s where the problem lies, if you were to ask the bot a question slightly out of its curriculum, it would fail tragically, regardless of how good the NLP system behind the bot is.
Here’s a quick example,
Imagine you own a flower shop. On your website, you have a chatbot that runs suggestions to your customers based on various questions that they might have, so you have fed the bot with the following FAQs:
Now suppose a customer comes to your website and just asks, “What flowers do I buy?”
Based on the NLP system running in the background, it might provide an error message, or it displays those pre-determined answers stated above based on a probability score.
Had this conversation happened in person, the florist may follow up with more questions like “For what occasion?”
Adding the functionality to segregate Intent and Entity in your chatbot FAQ's solves this issue. It gives your chatbot the ability to pose intelligent questions to draw more information from a user instead of resorting to fallback responses.
What are intents and entities in NLP?
Let’s go back to the example of the flowers.
“What flowers do I buy for my birthday?”
On looking at this statement, we can understand two basic things:
The former is the Intent, the latter, Entity. They more or less are the building blocks for most queries. Now, if you run a gift shop, you might not just be selling flowers; you might also sell gift cards, chocolates, cakes, and much more. In that case, you would want to add two or more Entities with the Intent of buying.

To break it down further, let’s define a few terms.
In the graphic above, we can understand that the user’s intention is to read the news.
What are utterances in a chatbot?
Utterances in chatbots are the inputs that the users provide to your chatbot, either through text or audio messages. Your chatbot will derive intents and entities from these utterances.
For the purpose of training your chatbot to derive intents and entities from your users’ messages with a greater degree of accuracy, you would need to have a range of different example utterances for each and every intent.
Chatbot development environments tend to use sample utterances as the training data that is used to construct a model used that is used to detect intent and entities.
How are intents and entities used in a chatbot?
After integrating these scenarios into Chatbot intents and entities, we now open up the possibility of having a more human-like interaction between a Customer and a Bot.
Take this interaction as an example:
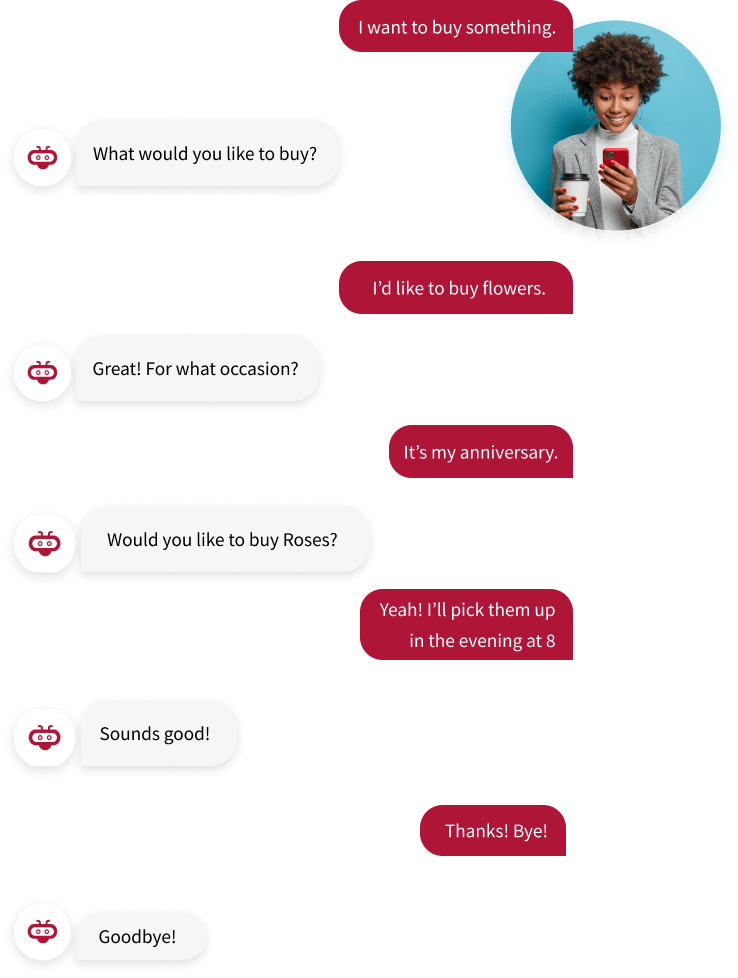
In this example, the customer tells the bot their Intent. The bot keeps asking questions to determine the Entities associated with this Intent, effectively helping them place an order tailored to their needs.
A chatbot will identify intents from every utterance and carry them across multiple messages so that it knows what the user wants to achieve from the conversation. An intent is basically the goal or aim that the user or customer has in mind when they are typing in their questions as utterances. The entity modifies user intent in the chatbot. These entities are tied to knowledge repositories so that there is a greater degree of personalization and accuracy in responses on user search. Chatbots can make use of the entities that they pull from the users’ utterances that they send to the bot and then make use of these entities to add values to the search intent. Intents and entities are especially important in customer service chatbots because your customers are already stressed and they need to get the right answers quickly in the most convenient way possible.
What are the 2 types of entities in NLP?
The two types of entities in a chatbot are:
What is the difference between intent and entity?
The difference between intent and entity is that the intent is the goal that your user has when they’re sending a message to your chatbot, while the entity is the modifier that your user makes use of to describe their issue.
The intent is an action that the user wants to perform and the entity is a keyword that you want to be extracted from the user’s utterance.
Why use an intent-based chatbot?
An intent-based chatbot offers several benefits in terms of the end user experience.
How to design your chatbot considering intent and entities?
One of the 1st steps a UX designer must focus on while working on a chatbot is picking up common customer questions.
An easy way of going about it is by collecting data from customer queries through calls, live chat, or other channels. However, at times the language is not concrete enough for humans to understand, let alone chatbots.
Here are 2 versions (or inputs) of the same situation-
Here, the first is clear and direct, while the second expects the chatbot to understand the underlying customer intent, which is to look for a nearby highly-rated Italian restaurant.
The entity that the chatbot picks could be ‘restaurant,’ ‘dinner,’ ‘take <noun pronoun=""> out,’ and respond accordingly.</noun>
Another example could be something like this-
Now, the second input suggests that the customer is looking for an iPhone repair shop. The entity in this example would be ‘broken,’ and what do you do with something broken? You have to fix it.
Therefore, you've got to design the structure to support the same. So, NLP is training and designing UI for chatbots to understand the intent and context of the conversation, which will further help conversation designers create the dialogue flow and relevant content.
Let’s look at intent, layout, and entity identification from the customer’s perspective. What is it that customers, or people generally do when they want to express their feelings, emotions, intentions, or requirements?
As we’ve mentioned above, customers either state their requirements directly or they beat around the bush. They can either ask questions, use exclamations, or keep browsing with the available options that the chatbot provides. They do what they feel is expressive enough and don’t call for extra effort unless incredibly frustrated.
So, how do you go about the design for the chatbot? Of course, it’s easy to understand what the customer is looking for when they directly state it, but even human inputs can be vague and outrageously confusing.
So, when you’re running a business and want to build a bot. You have 2 options.
You can either:
The first one is a red flag for your business. However, the second one is a powerful tool to bet your money on, and why not? Machines aren’t unruly anymore.
There’s a common misconception that devices work in a systematic order yet unintelligent manner. Perhaps, this was the case back in the 50s but not today.
The advancements in technology and NLP are enabling chatbots to compete with humans in the most technically challenging and intellectual fields.
This is the kind of intelligence and design that’s inbuilt in chatbots. It trains them to understand customer intent in a conversation.
We’re building a conversational interface that’s helping machines train themselves to interact with humans. Thanks to neural networks, bots are now intelligent enough to collect customer queries, provide efficient customer solutions, figure out what answers they don’t have, and train themselves to learn and bridge the gap. This training is making chatbots contextually more aware and emotionally robust, and intelligent. And the overall design works well for business.