What are loss functions?
The loss function is the function that computes the distance between the current output of the algorithm and the expected output. It’s a method to evaluate how your algorithm models the data. It can be categorized into two groups. One for classification (discrete values, 0,1,2…) and the other for regression (continuous values).
The loss function is used to measure how far off an estimated value is from its actual, true value. It maps decisions to their associated costs. Loss functions are not fixed. According to the goal that has to be met, and the task that needs to be accomplished, the loss functions change.
Essentially, a loss function can help you get an idea of how accurate the model’s decisions are.
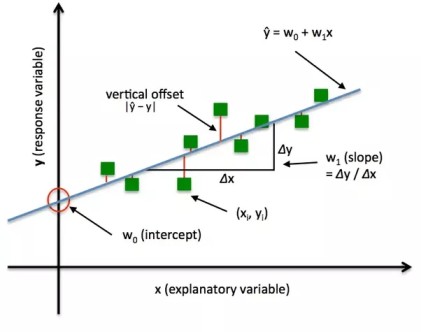
What is the loss function in a neural network?
The Loss Function is one of the important components of Neural Networks. Loss is nothing but a prediction error of Neural Net. And the method to calculate the loss is called Loss Function.
In simple words, the Loss is used to calculate the gradients. And gradients are used to update the weights of the Neural Net. This is how a Neural Net is trained.
What are the commonly used loss functions to train a Neural Network?
- Content
- Cross-entropy
- Log loss
- Exponential Loss
- Hinge Loss
- Kullback Leibler Divergence Loss
- Mean Square Error
- Mean Absolute Error
- Huber Loss
What are the most essential loss functions?
Mean Squared Error
MSE loss is used for regression tasks. As the name suggests, this loss is calculated by taking the mean of squared differences between actual(target) and predicted values.
Binary Crossentropy
BCE loss is used for the binary classification tasks. If you are using BCE loss function, you just need one output node to classify the data into two classes. The output value should be passed through a sigmoid activation function and the range of output is (0 – 1).
Categorical Crossentropy
When we have a multi-class classification task, one of the loss function you can go ahead is this one. If you are using CCE loss function, there must be the same number of output nodes as the classes. And the final layer output should be passed through a softmax activation so that each node output a probability value between (0–1).
Sparse Categorical Crossentropy
This loss function is almost similar to CCE except for one change. When we are using SCCE loss function, you do not need to one hot encode the target vector.
Likelihood loss
The likelihood function is also relatively simple, and is commonly used in classification problems. The function takes the predicted probability for each input example and multiplies them. And although the output isn’t exactly human-interpretable, it’s useful for comparing models.
Log loss
Log loss is a loss function also used frequently in classification problems, and is one of the most popular measures for Kaggle competitions. It’s just a straightforward modification of the likelihood function with logarithms.
Hinge Loss
The Hinge loss function was developed to correct the hyperplane of SVM algorithm in the task of classification. The goal is to make different penalties at the point that are not correctly predicted or too closed of the hyperplane.
How to minimize losses?
At its core, a loss function is a measure of how good your prediction model does in terms of being able to predict the expected outcome(or value). We convert the learning problem into an optimization problem, define a loss function and then optimize the algorithm to minimize the loss function.
An optimization problem seeks to minimize a loss function. An objective function is either a loss function or its negative (in specific domains, variously called a reward function, a profit function, a utility function, a fitness function, etc.), in which case it is to be maximized.
What is the difference between loss function and objective function?
The loss function (or error) is for a single training example, while the cost function is over the entire training set (or mini-batch for mini-batch gradient descent). Therefore, a loss function is a part of a cost function which is a type of an objective function.
Loss function is usually a function defined on a data point, prediction and label, and measures the penalty. For example:
- square loss l(f(xi|θ),yi)=(f(xi|θ)−yi)2, used in linear Regression
- hinge loss l(f(xi|θ),yi)=max(0,1−f(xi|θ)yi), used in SVM
- 0/1 loss l(f(xi|θ),yi)=1⟺f(xi|θ)≠yi, used in theoretical analysis and definition of accuracy
Cost function is usually more general. It might be a sum of loss functions over your training set plus some model complexity penalty (regularization). For example:
- Mean Squared Error MSE(θ)=1N∑Ni=1(f(xi|θ)−yi)2
- SVM cost function SVM(θ)=∥θ∥2+C∑Ni=1ξi (there are additional constraints connecting ξi with C and with training set)
Objective function is the most general term for any function that you optimize during training. For example, a probability of generating training set in maximum likelihood approach is a well defined objective function, but it is not a loss function nor cost function (however you could define an equivalent cost function). For example:
- MLE is a type of objective function (which you maximize)
- Divergence between classes can be an objective function but it is barely a cost function, unless you define something artificial, like 1-Divergence, and name it a cost
Error function - Backpropagation; or automatic differentiation, is commonly used by the gradient descent optimization algorithm to adjust the weight of neurons by calculating the gradient of the loss function. This technique is also sometimes called backward propagation of errors, because the error is calculated at the output and distributed back through the network layers.