What is sentiment analysis?
Sentiment analysis is a process of analyzing a piece of text and identifying opinions and judgments. This procedure shows you whether the sentiment of a piece of text is positive, negative, or neutral.
Natural Language Processing (NLP) and machine learning are used in unison to score the sentiments for categories, topics, or entities in a phrase. Along with ML and NLP, other kinds of data analysis techniques are also used to process and analyze the raw text and pull objective quantitative results from it.
Sentiment Analysis is also known as opinion mining or emotion AI. It can be used to transform unstructured text from social media, news, forums, blogs, etc., into structured data.
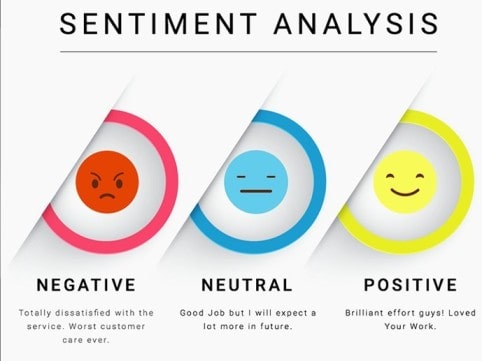
What is the purpose of sentiment analysis?
The purpose of sentiment analysis is to allow companies to understand the market’s sentiments regarding their brands or products. It is rather important because it enables brands to understand how their customers and prospective customers feel about them.
Understanding customer sentiments allows businesses to make higher quality and more informed decisions.
When humans perform sentiment analysis, the results tend to be very subjective. It depends on people’s personal experiences, their thoughts, beliefs, and many other factors. When you use an automated sentiment analysis system, your organization gets to to apply the same, uniform criteria to all your data, thus improving accuracy, creating consistency, and helping you gain better results.
Sentiment analysis helps you avoid PR nightmares. If someone told you that all publicity is good publicity, they’re either lying or they don’t know better. Bad publicity is certainly a thing, and you want to take care of it sooner rather than later. Not making use of sentiment analysis could result in your brand getting damaged substantially.
What is sentiment analysis used for?
Sentiment analysis is used by organizations extensively to conduct market research. It is also used to improve social listening by analyzing the sentiment that the market displays towards the brand on social media.
It is also useful to understand the underlying emotions in customer feedback and improve the customer experience based on that. It’s great for understanding the Voice of the Customer
Sentiment analysis is deployed in intelligent customer service chatbots to understand the customer’s emotional state and deliver an appropriate response. Here’s why that is critical: Imagine that a customer sends a message in a tone that clearly indicates frustration, and gets a reply in a chipper, happy tone. That would annoy the customer even further. In this situation, a chatbot should be able to understand the customer’s emotional state, empathize with them
Sentiment analysis also helps you understand the Voice of the Employee by analyzing employee surveys, Glassdoor reviews, etc.
Sentiment analysis makes it possible for businesses to sort through massive quantities of data automatically, in an efficient and cost effective manner. It can also help identify urgent and vital issues in real-time, making it possible for you to take immediate action before things go bad.
How does sentiment analysis work?
In sentiment analysis, text is first broken down into topics and then scores are assigned to the topics, on a pre-decided scale.
After that, the system either uses each score individually to evaluate specific parts of the text or it adds up all the scores for the topics.
What are the types of sentiment analysis?
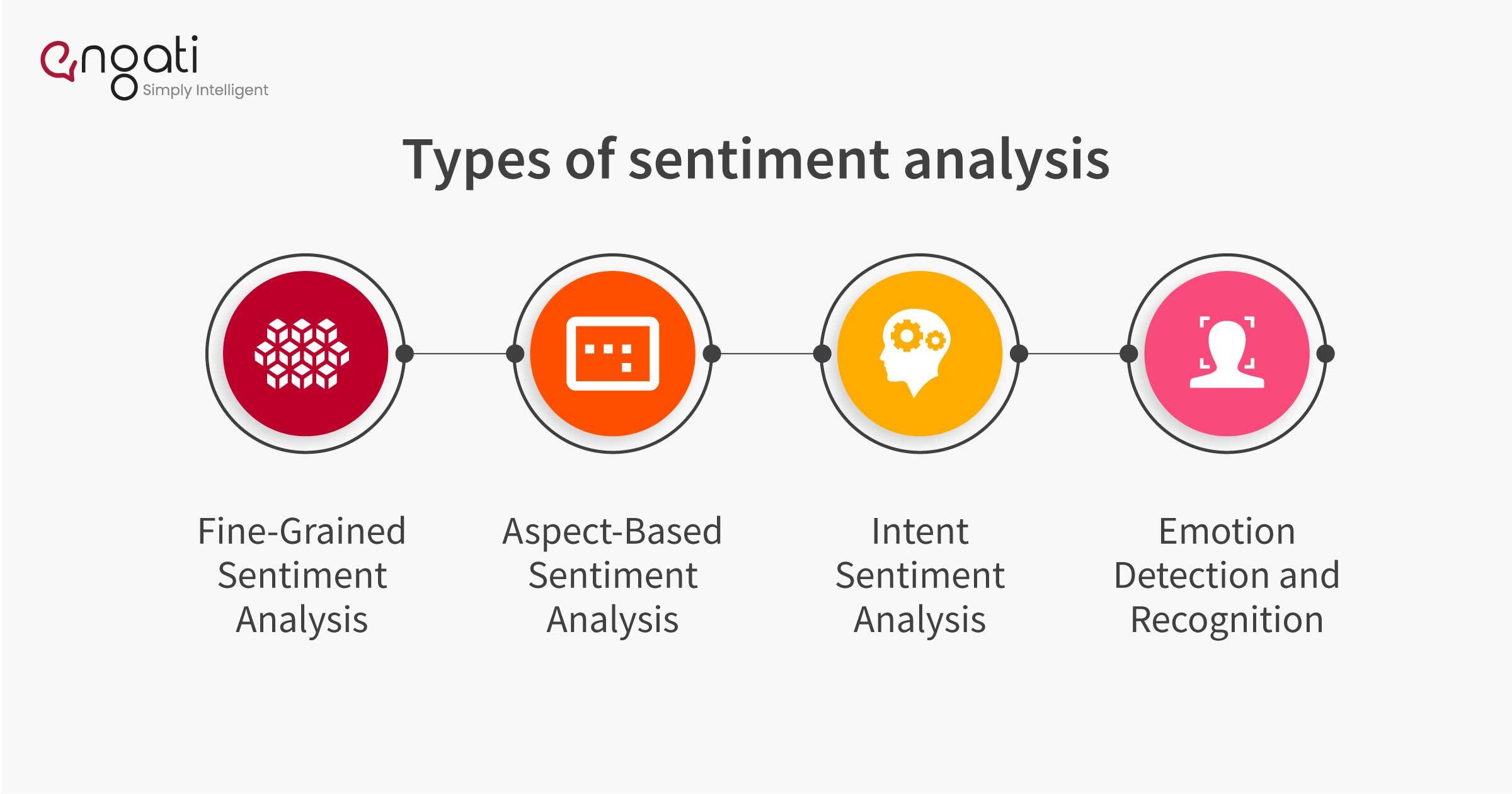
Here are the most popular types of sentiment analysis:
Fine-Grained Sentiment Analysis
Most sentiment analysis systems employ fine-grained sentiment analysis. It concentrates on the polarity of an opinion. Rather than rating sentiments to just be positive, negative, or neutral, it segments them further into very positive, somewhat positive, neutral, somewhat negative, and very negative.
It can pull this data from product reviews, surveys, etc.
The polarity detected can be tied to emotions as well.
Very positive = love
Somewhat positive = acceptance
Neutral = indifference
Somewhat negative = anxiety
Very negative = anger
Aspect-Based Sentiment Analysis
Instead of just looking at polarity, aspect-based sentiment analysis allows you to consider the sentiment regarding specific aspects, features, or elements of your product or service.
It helps you understand how your customers feel about specific attributes of your offerings.
It is essentially a text analysis method that classifies sentiments according to aspects and detects the sentiment that is attributed to each one. It also allows organizations to automatically analyze enormous amounts of data in detail, thus saving money and time and allowing teams to focus on more important tasks.
Intent Sentiment Analysis
This is about understanding the intent that lies behind a message. Is it an opinion? Does it show appreciation? Is it a complaint, a question, or a suggestion?
However, since it is difficult to do this without context, this is still a concept and is not practically used yet. It involves making use of Long Short Term Memory (LSTM) algorithms to categorize text into various LSTMs model sentences as chain of forget-remember decisions based on context
Emotion Detection and Recognition
This relies more on algorithms and uses lexicons and machine learning to understand customer emotions. But it is not just limited to simply showing you the emotion, it helps you understand why customers feel how they do.
The majority of emotion detection systems make use of lexicons (lists of words along with the emotions that they convey) or complex machine learning algorithms.
But just using lexicons might not always be the best idea. This is because people tend to express emotions in various ways. Words that typically convey negative emotions could also be used to expression positive ones. For example, In the phrase ‘It makes me sick’, the word ‘sick’ conveys a negative emotion, but in the phrase ‘This is sick! I love it’, the word ‘sick’ conveys a positive emotion.