Knowledge Engineering
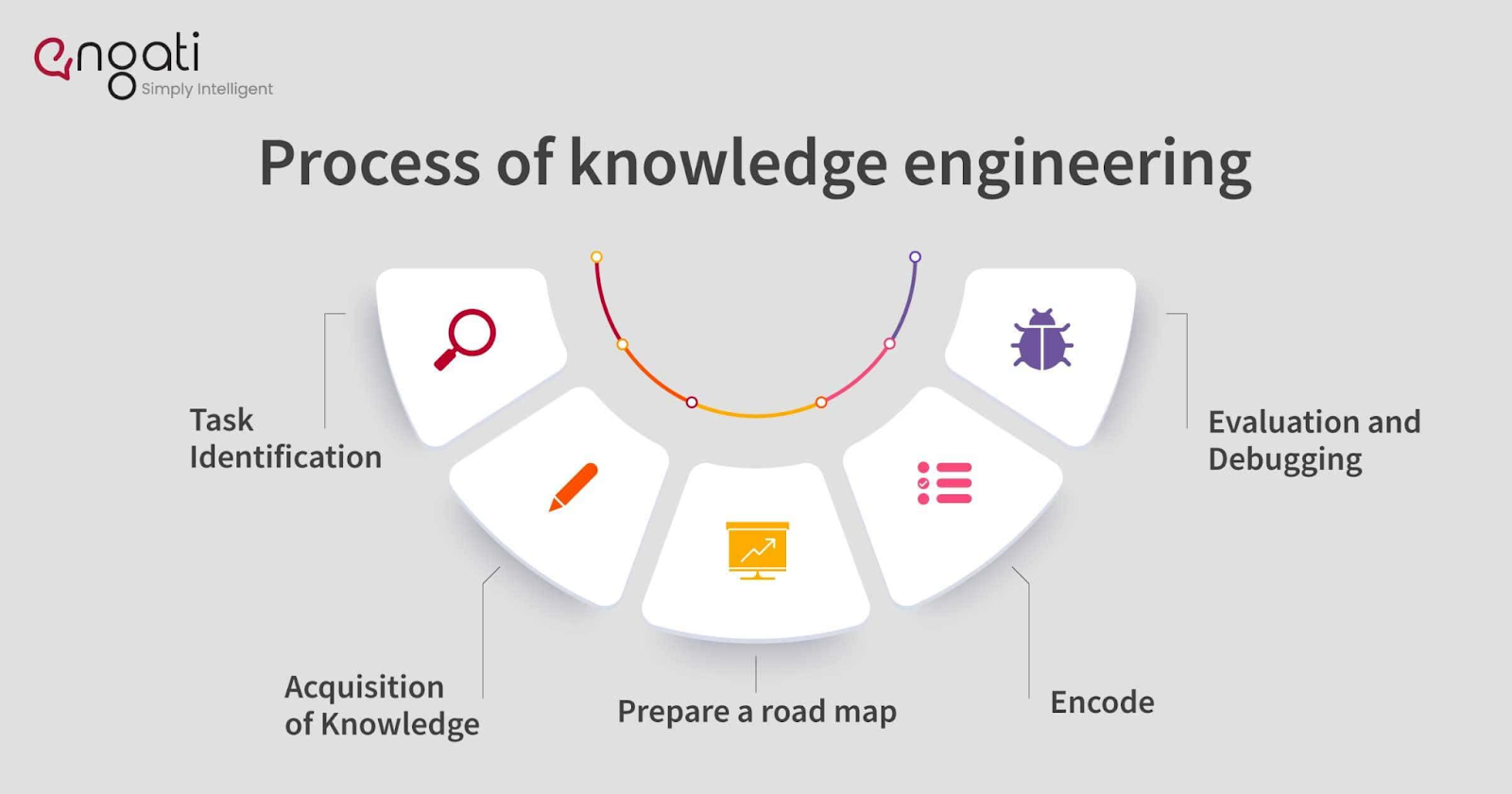
What is knowledge engineering?
Knowledge engineering is a branch of artificial intelligence (AI) that develops rules that are applied to data to imitate the thought process of a human who is an expert on a specific topic. This field of artificial intelligence attempts to emulate the judgment and behaviour of a human expert in a particular field.
In its initial form, knowledge engineering focused on the transfer process; transferring the expertise of a problem-solving human into a program that could take the same data and make the same conclusions.
It was determined that transfer processing had its limitations, as it did not accurately reflect how humans make decisions. It did not consider intuition and gut feeling, known as analogous reasoning and nonlinear thinking, which often may not be logical. Another reason why the transfer approach was not successful was that the systems did not have the collateral information (information that is not central to a specific issue or field but is still useful in making judgements and decisions) that human specialists and experts had.
Today, knowledge engineering uses a modelling process that creates a system that touches upon the same results as the expert without following the same path or using the same information sources.
The goal of knowledge engineering is for it to be implemented into software that will make decisions that human experts would, such as financial advisors.
Knowledge engineering is already being used in decision support software and it is expected that at some point it will be used to make better decisions than human experts. This is the technology that is used to build expert systems that assist humans with issues pertinent to the field of knowledge for which they are programmed. Knowledge engineering systems might also make use of machine learning to make it possible for these systems to learn from their experiences like the way humans learn.

What is the knowledge engineering process?
Knowledge Engineering for different domains is different but it follows, the same set of procedures to create expert systems.
1. Task Identification
This is the first step in the knowledge engineering process where the task to be performed is defined. In a domain, a specific problem or a combination of several problems would be taken. This task must be realistic and the subject matter expert needs to have a clear idea of what it is so that further process can be carried out.
2. Acquisition of Knowledge
Once the problem is well defined then the next step is to gather relevant knowledge and information about the problem. For some problems,2 standard data is used that must be collected, for example, a problem on heat exchanger requires the standard steam table data at x temperature and y pressure what will be the value of enthalpy.
3. Prepare a road map
Once the goal and knowledge base are available the next step is to get the roadmap ready by breaking the goal down into small steps by questionnaires and relevant knowledge base. Here the subject matter expert puts his thoughts on how he would make decisions and what parameters would be considered at all stages. There could be several ways to solve some problems, and all should be considered.
4. Encode
Now it’s time to convert this knowledge into computer language. Here the knowledge is encoded by using different functions as well as in some cases, for a specific task, the algorithm is used to create a model. These models are able to make decisions based on available parameters as an expert does, surely the model must be trained and tested on a sufficient amount of data.
5. Evaluation and Debugging
In the process of creating an expert system, at each step, the model should be evaluated and debugged and then added to workflow. Once all small tasks are evaluated, they are assembled to create one whole expert system. This system is again evaluated on similar problems and Debugged if any issue is there.
What are the benefits of knowledge engineering?
In today’s world, people do not want to spend time waiting for a human expert to be available to resolve their issues. They want answers to their queries instantly and that’s where expert systems built with knowledge engineering can shine.
Knowledge engineering, the process of designing, building, and maintaining knowledge-based systems, offers several benefits:
Efficient Problem Solving: Knowledge engineering enables the capture and organization of expertise and domain knowledge, allowing for more efficient problem-solving. By encoding human expertise into computer systems, it becomes possible to automate complex tasks and decision-making processes.
Decision Support: Knowledge-based systems can provide decision support by offering recommendations or suggesting actions based on the rules and knowledge encoded within them. This can assist professionals in various fields, from medicine to finance, in making informed decisions.
Consistency and Accuracy: By codifying knowledge into a structured format, knowledge engineering helps ensure consistency and accuracy in decision-making processes. Computers can apply rules and knowledge without bias or variation, leading to more reliable outcomes.
Scalability: Once knowledge is encoded into a system, it can be easily scaled to handle large amounts of data and diverse problem domains. This scalability is particularly beneficial in domains where expertise is in high demand or where there is a need to process vast amounts of information.
Knowledge Preservation: Knowledge engineering allows for the preservation and dissemination of expertise, even in fields where experts may be scarce or where knowledge is at risk of being lost over time. By capturing knowledge in a digital format, it can be stored, shared, and reused as needed.
Continuous Improvement: Knowledge-based systems can be updated and refined over time to reflect new insights, discoveries, or changes in the domain. This allows for continuous improvement and adaptation to evolving circumstances, ensuring that the system remains relevant and effective.
Cost Reduction: In many cases, knowledge engineering can lead to cost reductions by automating tasks that would otherwise require human expertise. This can result in savings in terms of time, labour, and resources.
Overall, knowledge engineering plays a crucial role in leveraging human expertise and domain knowledge to develop intelligent systems that can assist in decision-making, problem-solving, and knowledge management across various domains.