What is Explainable AI?
At the core of Explainable AI lies the question:
How can you trust something you don’t understand?
Explainable AI also known as XAI, is the practice using a set of techniques and approaches to design AI models in such a manner that the model’s output and decision-making process is more transparent, interpretable and understandable to humans.
Why is Explainable AI important?
Picture this:
Confronted with a complex problem, you wander far and wide seeking a solution. Suddenly, before you, appears a seemingly wise entity claiming to know it all.
You present your problem, and the entity effortlessly answers. While the solution has some logic to it, you still have your doubts. Just as you’re about to put forth your concerns, the entity vanishes into the void.
What would you do?
Blindly accept and implement the solution given the risk and high stakes?
Or try to make sense of the solution before blindly accepting and implementing it.
If only there was a way you could ask the entity how it reached its conclusion.
Artificial Intelligence has been shaking things up ever since its arrival. To top it all off, this technology is only growing.
Especially in industries such as healthcare, autonomous vehicles, finance and government where one wrong move could result in severe consequences, comprehending how decisions are made by technology is absolutely crucial and non-negotiable.
The entity above referred to AI, which promises solutions, but isn’t always capable of informing us how it got there.
And this is where explainable AI comes in.
Explainable AI seeks to resolve the uncertainty of trusting something beyond one’s understanding by explaining it to them, hence validating the technology and assuring the people who use it.
The case of Amazon’s AI: A sexist system
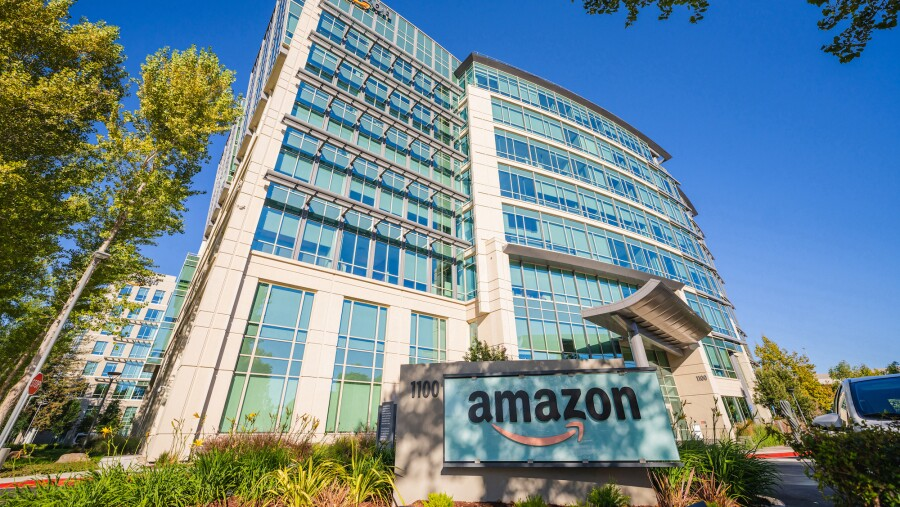
The biggest e-commerce giant, Amazon has always been keen on automation.
Back in 2014, Amazon paved the way for utilising AI for automating its hiring process, where the AI system would review job applications, rate resumes and output the top ‘talent’.
It was only a year after when they realised: This system was sexist.
When it came to technical posts such as software developer jobs, the AI preferred male candidates and penalised the words “women’s”, going as far as completely downgrading candidates from certain “all women’s” colleges.
Why?
Since the system was trained on the resumes of candidates hired by the company over a 10 year period, which mostly belonged to men reflecting the male dominated tech industry. Hence, it rewarded male-related terms while penalising term “women” and other related terms
If only this information was clear when the automation system was put in place, measures could’ve been taken to mitigate the bias, fix the AI, or reconsider its implementation.
How Explainable AI works
Conventional AI vs. Explainable AI
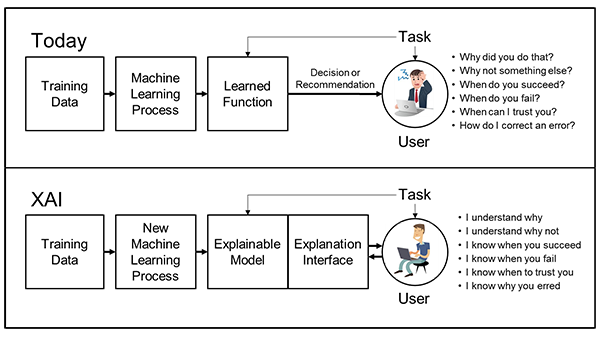
As Explainable AI refers to a set of techniques and approaches, these are explained with respect to each of its objectives below:
Methods to ensure transparency
Transparency in AI refers to the extent to which the decision-making processes of AI models and their inner workings are accessible, clear, and understandable to humans.
- Rule-Based Models rely on explicit rules to generate predictions, making their decision logic straightforward and transparent.
- Feature Importance Analysis utilise techniques like SHAP (SHapley Additive exPlanations) and LIME (Local Interpretable Model-Agnostic Explanations) which quantify feature contributions, offering insights into feature significance. SHAP values provide detailed contributions, while LIME approximates behavior using simpler models.
- Visualization tools and techniques like Partial Dependence Plots illustrate feature-prediction relationships, and Individual Conditional Expectation (ICE) Plots show how individual predictions change with specific feature variations.
- Feature Attribution Methods like Integrated Gradients quantify feature impact on predictions, aiding transparency.
- Attention Mechanisms such as Neural network attention maps highlight vital input regions, revealing model focus on specific features.
- Ethical and Regulatory Compliance: Incorporating fairness metrics ensures unbiased and ethical model behavior.
Methods to ensure interpretability
Interpretability in AI refers to the ability of an AI model's logic, relationships between features, and factors influencing its prediction to be comprehensible and explainable to humans.
- Interpretable Models: Utilizing models like Decision Trees, Linear Regression, and Rule-Based Models that offer clear decision paths, simple relationships, and explicit decision rules.
- Prototype and Feature Relevance Analysis: Creating prototype instances representing categories to understand model-learned features and identifying influential features in predictions.
- Natural Language Explanations: Enhancing interpretability through explanations provided in natural language i.e. human-like explanation.
- Meta-Interpreters: Models like Rationale-Net generate explanations for complex models, acting as interpreters.
- Model Distillation: Training smaller models to mimic complex models' behavior results in a more interpretable model, simplifying understanding.
Methods to ensure understandability
Understandability refers to the quality of AI models' outputs, explanations, and decision-making processes being easily comprehensible to humans.
- Natural Language Explanations:
- Textual Descriptions: Provide insights into the reasoning behind specific decisions.
- Visualization tools that Utilize user-friendly visual formats to enhance data presentation.
- Counterfactual Explanations:
- Counterfactuals: Demonstrate alternative instances with varying outcomes, aiding understanding of input impact.
- Local Explanations: Focus on explaining individual predictions to enhance understanding at the instance level.
- Decision Understanding: Educate team members and others working with AI technologies.
Explainable AI Challenges and What’s next
Currently a challenge is Explainable AI is the predictability vs. interpretability payoff. Conventional AI models usually give more efficient, accurate solutions compared to their Explainable AI counterparts.
The future of Explainable AI involves refining techniques for its core conditions: transparency, interpretability, and understandability, and also tailoring them for specific domains.
More human-AI collaboration along with interdisciplinary research and education is required to enhance overall understanding, quality of AI decisions, which would encourage overall growth and applications of AI.