What is Apriori Algorithm?
Apriori algorithm is an influential algorithm that is generally used in the field of data mining & association rule learning. It is used to identify frequent itemsets in a dataset & generate an association based rule based on the itemsets.
Imagine you have a database about the items a customer purchases from the store. The Apriori algorithm helps to uncover interesting relationships & patterns in this data. It does that by finding the sets of items that occur together, frequently.
For e.g. the algorithm would discover that when a customer buys bread, they often end up buying butter & eggs as well. This indicates a strong association between these items. These associations help businesses to make decisions to improve sales, customer satisfaction, etc.
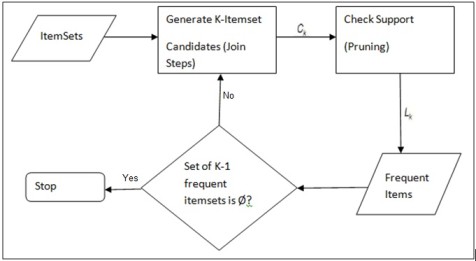
What are the steps of Apriori Algorithm in data mining?
The following are the main steps of the apriori algorithm in data mining:
- Set the minimum support threshold - min frequency required for an itemset to be "frequent".
- Identify frequent individual items - count the occurence of each individual item.
- Generate candidate itemsets of size 2 - create pairs of frequent items discovered.
- Prune infrequent itemsets - eliminate itemsets that do no meet the threshold levels.
- Generate itemsets of larger sizes - combine the frequent itemsets of size 3,4, and so on.
- Repeat the pruning process - keep eliminating the itemsets that do not meet the threshold levels.
- Iterate till no more frequent itemsets can be generated.
- Generate association rules that express the relationship between them - calculate measures to evaluate the strength & significance of these rules.
What are the advantages of Apriori Algorithm in data mining?
.webp)
After the steps of Apriori algorithm in data mining, it's advantages are as follows:
- Simplicity & ease of implementation
- The rules are easy to human-readable * interpretable
- Works well on unlabelled data
- Flexibility & customisability
- Extensions for multiple use cases can be created easily
- The algorithm is widely used & studied
What are the disadvantages of Apriori Algorithm in data mining?
Following are the disadvantages of the apriori algorithm in data mining:
- Computational complexity
- Time & space overhead
- Difficulty handling sparse data
- Limited discovery of complex patterns
- Higher memory usage
- Bias of minimum support threshold
- Inability to handle numeric data
- Lack of incorporation of context
How can we improve the Apriori Algorithm's efficiency?
Here are some of the methods how to improve efficiency of apriori algorithm -
- Hash-Based Technique: This method uses a hash-based structure called a hash table for generating the k-itemsets and their corresponding count. It uses a hash function for generating the table.
- Transaction Reduction: This method reduces the number of transactions scanned in iterations. The transactions which do not contain frequent items are marked or removed.
- Partitioning: This method requires only two database scans to mine the frequent itemsets. It says that for any itemset to be potentially frequent in the database, it should be frequent in at least one of the partitions of the database.
- Sampling: This method picks a random sample S from Database D and then searches for frequent itemset in S. It may be possible to lose a global frequent itemset. This can be reduced by lowering the min_sup.
- Dynamic Itemset Counting: This technique can add new candidate itemsets at any marked start point of the database during the scanning of the database.
What are the components of the Apriori algorithm?
There are three major components of the Apriori algorithm in data mining which are as follows.
- Support
- Confidence
- Lift
For example, you have 5000 customer transactions in a Zara Store. You have to calculate the Support, Confidence, and Lift for two products, and you may say Men's Wear and Women Wears.
Out of 5000 transactions, 300 contain Men's Wear, whereas 700 contain women's wear, and these 700 transactions include 250 transactions of both men's & women's wear.
1. Support
Support denotes the average popularity of any product or data item in the data set. We need to divide the total number of transactions containing that product by the total number of transactions.
Support (Men's wear)= (transactions relating MW) / (total transaction)
= 300/5000
= 16.67 %
2. Confidence
Confidence is the sum average of transactions/data items present in pairs/combinations in the universal dataset. To find out confidence, we divide the number of transactions that comprise both men's & women's wear by the total number of transactions.
Hence,
Confidence = (Transactions with men's & women's wear) / (total transaction)
= 250/5000
= 5%
3. Lift
It helps find out the ratio of the sales of women's wear when you sell men's wear. The mathematical equation of lift is mentioned below.
Lift = (Confidence ( Men's wear- women's wear)/ (Support (men's wear)
= 20/18
= 1.11
What are the applications of Apriori Algorithm in data mining?
Apriori Algorithm has picked up a pace in recent years and is used in different industries for data mining and handling.
Some fields where Apriori is used:
1. Medical
Hospitals are generally trashed with data every day and need to retrieve a lot of past data for existing patience. Apriori algorithm help hospitals to manage the database of patients without jinxing it with other patients.
2. Education
The educational institute can use the Apriori algorithm to store and monitor students' data like age, gender, traits, characteristics, parent's details, etc.
3. Forestry
On the same line as the education and medical industry, forestry can also use the Apriori algorithm to store, analyze and manage details of every flora and fauna of the given territory.
4. New Tech Firms
Tech firms use the Apriori algorithm to maintain the record of various items of products that are purchased by various customers for recommender systems.
5. Mobile Commerce
Big data can help mobile e-commerce companies to deliver an easy, convenient and personalized shopping experience. With the Apriori algorithm, the real-time product recommendation accuracy increases, which creates an excellent customer experience and increases sales for the company.