Let’s face it. Data is scattered all over the place in silos in an enterprise. "Starting from email servers, intranet, countless documents, reports, presentations, charts/graphs in various document repositories, ERP, internal systems, e.g., HRIS, databases, source code control system, release repositories, wikis, ticketing system, etc.", we can keep going and find how data is distributed in the enterprise.
Now let’s take a familiar situation. Say the CEO of a company wants to find everything about an employee. Someone, who recently presented a path-breaking paper at an internal conference. And he wants to put together a crack engineering team that can work with this employee on a futuristic project. Imagine the number of people who have to work on this query to get the most up-to-date information about this employee and present it to the CEO.
What if the CEO had follow-up questions or wanted more aggregate information?
"Behind the scenes", we will need many people with different skill sets to "find, read, summarise", and put together the best information that exists in numerous systems. As a matter of fact, it is very likely that hundreds of hours would be spent until they get enough information for the CEO to take the decision.
What this problem points to is the fact that there is no easy way for the CEO to directly access the information which is locked away in hundreds of data systems. Now let’s imagine the CEO has a chatbot through which he/she can ask a query in natural language and get all the answers he/she needs at whatever level it matters. This can be a powerful tool at the hands of everyone in an organization to get access to locked away transformed data in seconds and help in quick decision making. Plus it will be a big step to democratize data in the enterprise, which is sorely missing today. A problem that Engati is actively solving for CEOs and corporates.
How does the chatbot use data?
The chatbot is the interface through which the user will enter the queries in the language he/she is most familiar with. Behind the scenes, we will need a backend system that can translate this natural language query into a system-specific query. Then aggregate the query responses from multiple systems and respond in "text, image or voice.
Let’s take the first challenge. Translating the natural language query into a system-specific query. It is in the purview of the chatbot platform.
This is how data is scattered in an enterprise
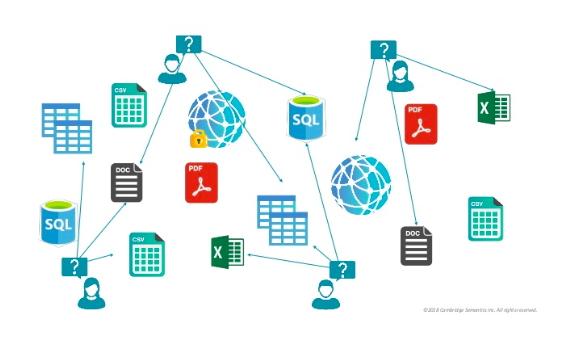
And this is what the chatbot needs to do the translation

This problem is not a simple one and involves many techniques. In fact, it involves technologies to come together with, such as NLP, NLU, NLG, Deep Learning, Reinforcement Learning. This is for them to be able to understand the natural language query. Therefore, they can translate it into a backend system-specific query.
As an example, let’s say we have the employee information in an RDBMS. We have to translate the user query into an equivalent SQL query. Hence, it will need a Deep Learning model to translate Natural Language to SQL.
We will explore this further in subsequent articles. For instance, what machine learning models and tools can we use to solve this problem in the best possible manner. For that matter, the team here at Engati is working towards solving the problem.
The future is here! Manage your data through a chatbot and revolutionize information processing for your business. Register with Engati today and take a free chatbot demo now!