What is a genetic algorithm?
A genetic algorithm (GA) is a search heuristic that is inspired by Charles Darwin’s theory of natural evolution. This algorithm reflects the process of natural selection where the fittest individuals are selected for reproduction in order to produce offspring of the next generation.

How do genetic algorithms work?
Genetic algorithms work by simulating the logic of Darwinian selection, where only the best are selected for replication. Over many generations, natural populations evolve according to the principles of natural selection and stated by Charles Darwin in The Origin of Species. Only the most suited elements in a population are likely to survive and generate offspring, thus transmitting their biological heredity to new generations.
Genetic algorithms are able to address complicated problems with many variables and a large number of possible outcomes by simulating the evolutionary process of “survival of the fittest” to reach a defined goal. They operate by generating many random answers to a problem, eliminating the worst and cross-pollinating better answers. Repeating this elimination and regeneration process gradually improves the quality of the answers to an optimal or near-optimal condition.
In computing terms, a genetic algorithm implements the model of computation by having arrays of bits or characters (binary string) to represent the chromosomes. Each string represents a potential solution. The genetic algorithm then manipulates the most promising chromosomes searching for improved solutions.
A genetic algorithm operates through a cycle of three stages:
- Build and maintain a population of solutions to a problem
- Choose the better solutions for recombination with each other
- Use their offspring to replace poorer solutions.
Genetic Algorithm coding
Each individual of a population represents a possible solution to a given problem. Each individual is assigned a “fitness score” according to how good a solution to the problem it is.
A potential solution to a problem may be represented as a set of parameters. For example, if our problem is to maximize a function of three variables, F(x; y; z), we might represent each variable by a 10-bit binary number (suitably scaled). Our chromosome would therefore contain three genes, and consist of 30 binary digits.
Fitness function
A Fitness function must be specific for each problem to be solved. Given a particular chromosome, the fitness function returns a single numerical merit proportional to the utility of the individual that chromosome represents.
Reproduction
During the reproductive phase of the GA, individuals are selected from the population and recombined. Parents are selected randomly from the population using a scheme which favors individuals with higher fitness scores.
Having selected two parents, their chromosomes are recombined, typically using the mechanisms of crossover and mutation:
- Crossover takes two individuals, and cuts their chromosome strings at some randomly chosen position, to produce two “head” segments, and two “tail” segments. The tail segments are then swapped over to produce two new full length chromosomes. The two individual each inherit some genes from each parent.
- Mutation is applied to each child individually after crossover. It randomly alters each gene with a small probability (typically 0.001).
If the GA has been correctly implemented, the population will evolve over successive generations so that the fitness of the best and the average individual in each generation increases towards the global optimum.
What are applications of genetic algorithms?
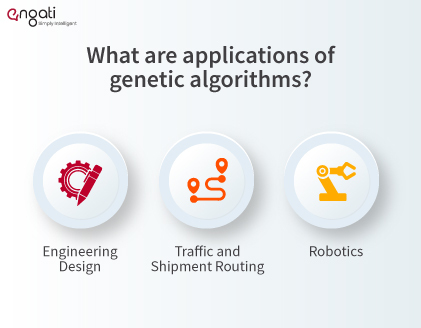
1. Engineering Design
Engineering design has relied heavily on computer modeling and simulation to make design cycle process fast and economical. Genetic algorithm has been used to optimize and provide a robust solution.
2. Traffic and Shipment Routing
This is a famous problem and has been efficiently adopted by many sales-based companies as it is time saving and economical. This is also achieved using genetic algorithm.
3. Robotics
The use of genetic algorithm in the field of robotics is quite big. Actually, genetic algorithm is being used to create learning robots which will behave as a human and will do tasks like cooking our meal, do our laundry etc.
What are the types of genetic algorithms?
There are four types of genetic algorithms:
Generational Genetic Algorithm (GGA)
In a generational genetic algorithm, if the population size is P, then P offspring are created and mutated. The replacement strategy replaces all the parents with their offspring. Now there is no overlap between the pre-existing population and the new one, so there is 0 elitism. The generation gap is zero.
The GGA makes use of the tournament selection method to randomly select two parents from the population to create one offspring. The process is repeated till the number of offspring matches the size of the pre-existing population. All the members of the pre-existing population are then wiped out.
Steady state (µ + 1)-Genetic Algorithm (SSGA)
The steady state genetic algorithm picks two parents to generate an offspring. Then it replaces the worst fit individual in the population with offspring. An SSGA is better than a GGA because it only makes one function evaluation per child on each cycle while a GGA needs to make P (where P is the population size) function evaluations on every cycle. Because of this, function evaluations are only counted while making comparisons between SSGAs and GGAs.
Steady-Generational (µ, µ)-Genetic Algorithm (SGGA)
The steady-generational genetic algorithm first picks the two parents to generate the offspring, and then instead of the offspring replacing the parents or the worst-fit individual, it just replaces a random individual from the population that isn’t the best fit. The SGGA only makes two function evaluations on each cycle.
(µ + µ)-Genetic Algorithm
The (µ + µ)-Genetic Algorithm uses binary tournament selection to randomly select two parents, create an offspring, and then add the offspring to a child population until the child population is of the same size as the original population.
It then creates a new population that is made up of the original population and the child population and picks the top individuals from this new population till the population is of the same size as the original population. This ensures that the algorithm composes s a population of the most fit individuals from two generations of individuals.